Ai Synthesis Ota Filter
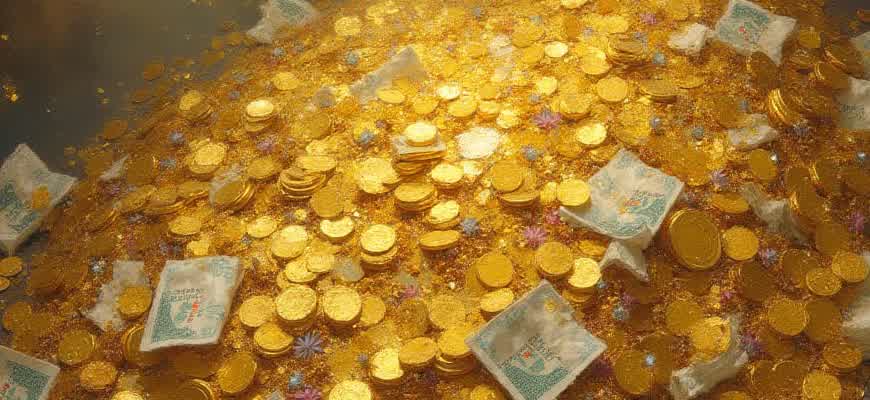
The integration of Artificial Intelligence (AI) in signal processing has revolutionized various fields, particularly in optimizing the synthesis of audio and visual data. AI-driven synthesis allows for the creation of complex patterns and structures from simpler, raw input. One of the key applications of AI in this domain is Ota filtering, a crucial process for refining and enhancing the quality of synthesized outputs.
Ota filtering leverages advanced machine learning algorithms to isolate and remove noise, distortions, or irrelevant information from a signal. This is essential for improving the fidelity of synthesized content, especially in environments with a lot of interference or data corruption.
- Purpose of AI Synthesis: Improve data processing efficiency and accuracy.
- Role of Ota Filtering: Enhance signal clarity by eliminating unwanted noise.
- Applications: Used in audio processing, image generation, and video enhancement.
"AI synthesis combined with Ota filtering represents the future of clean, high-quality data processing in real-time applications."
Key advantages of using AI synthesis with Ota filtering:
Advantage | Explanation |
---|---|
Increased Precision | AI models can predict and adjust for minor imperfections in data synthesis. |
Reduced Noise | Ota filtering minimizes the impact of extraneous noise in signals. |
Enhanced Speed | AI algorithms process data faster, optimizing real-time performance. |
AI-Driven Synthesis in OTA Filters: A Practical Guide for Implementation
AI-powered synthesis in operational transconductance amplifier (OTA) filters offers a novel approach to optimizing signal processing systems. By leveraging artificial intelligence techniques, these filters can dynamically adjust to varying conditions and requirements, improving performance over traditional fixed-parameter designs. This guide explores how AI can be applied to OTA filter synthesis, covering the key concepts, implementation steps, and real-world applications.
In this context, the AI approach allows for the creation of more adaptive and efficient filter circuits, which are crucial in a range of industries such as telecommunications, audio engineering, and medical devices. Using AI, engineers can design filters that automatically tune to the best possible configuration, optimizing parameters like frequency response, noise rejection, and signal integrity.
Key Advantages of AI-Based OTA Filter Synthesis
- Dynamic Adaptation: AI enables real-time adjustments, ensuring optimal performance under varying operational conditions.
- Increased Efficiency: By automating the design process, AI minimizes manual tuning, saving time and reducing errors.
- Improved Accuracy: AI algorithms can predict and adjust filter parameters with precision, enhancing the overall system performance.
Practical Steps for AI-Driven OTA Filter Design
- Data Collection: Gather a dataset of operational conditions, including desired frequency responses, noise characteristics, and system limitations.
- AI Model Training: Train a machine learning model using the collected data to predict the best filter parameters for given input conditions.
- Filter Design Optimization: Use the trained model to synthesize OTA filter designs, fine-tuning the architecture and component values.
- Testing and Validation: Implement the AI-synthesized filter in a prototype system, testing its real-world performance against expected benchmarks.
Key Considerations for Successful Application
Consideration | Explanation |
---|---|
Model Complexity | Ensure the machine learning model is complex enough to capture relevant parameters, but not overly complicated to avoid overfitting. |
Hardware Constraints | Take into account the limitations of the physical hardware when designing AI-driven filters, ensuring compatibility with real-world systems. |
Real-Time Processing | AI models must be efficient enough to work in real-time, particularly for applications requiring immediate adjustments. |
"AI synthesis in OTA filter design offers a transformative way to meet the growing demands for adaptive and high-performance signal processing systems."
How AI-Driven OTA Filters Enhance Real-Time Signal Processing
AI-based On-the-Air (OTA) filters have revolutionized the way signal processing is optimized in real-time communication systems. These intelligent filters, powered by machine learning algorithms, offer a dynamic approach to signal analysis and optimization, adapting quickly to changes in the environment and improving the quality of received data. By continuously learning from incoming signals, the AI synthesis technology can filter out unwanted noise and interference while enhancing signal clarity, ensuring that data transmission remains reliable and efficient.
The key advantage of AI-driven OTA filters is their ability to process signals on-the-fly, without the need for external adjustments. This real-time capability is critical for applications that require continuous data streams, such as wireless communication, IoT devices, and automotive systems. By using advanced predictive models, these filters can anticipate and correct potential distortions before they impact the system's performance, allowing for smoother and faster data exchange.
Key Features of AI-Optimized OTA Filters
- Real-Time Adaptation: AI algorithms continuously adjust filter parameters based on incoming signal characteristics.
- Noise Reduction: Machine learning techniques identify and suppress background noise, improving signal clarity.
- Predictive Analysis: AI models anticipate signal distortions and apply corrective measures before they affect communication.
- Efficiency in Resource Utilization: AI filters optimize power and processing resources by adapting to changing signal environments.
How AI Synthesis Works in OTA Filtering
The AI synthesis process involves several key stages:
- Signal Capture: Raw data is captured by sensors or antennas.
- Signal Classification: AI algorithms classify the signal type (e.g., noise, interference, or valid data).
- Adaptive Filtering: Based on the classification, the AI adjusts the filter settings to optimize signal quality.
- Real-Time Adjustment: The AI continuously refines its approach as the signal environment changes.
AI-driven OTA filters provide unparalleled flexibility and precision in signal processing, ensuring high-quality communication even in challenging environments.
Performance Metrics
Metric | Standard OTA Filters | AI-Optimized OTA Filters |
---|---|---|
Noise Reduction Efficiency | Moderate | High |
Real-Time Adaptation | Low | High |
Power Consumption | Higher | Optimized |
Understanding the Key Features of AI-Driven OTA Filter Technology
AI-based OTA filter technology integrates advanced machine learning techniques to optimize the performance of communication systems. Unlike traditional filters, which rely on static algorithms, these AI filters continuously adapt to the changing conditions of the signal environment. By leveraging deep learning models, AI filters can intelligently distinguish between noise, interference, and valid data, ensuring a clear and reliable signal. This dynamic approach enables better signal quality and more efficient resource usage, even in complex, high-traffic networks.
The most significant features of AI-enhanced OTA filters revolve around their ability to process signals in real-time, adapting and correcting distortions as they occur. Through constant learning, these filters can improve over time, providing increasingly refined signal processing that is crucial for modern wireless communication. This technology is especially beneficial for applications such as 5G networks, IoT devices, and autonomous systems, where uninterrupted and high-quality signal transmission is essential.
Core Features of AI-Optimized OTA Filters
- Continuous Adaptation: AI algorithms adjust filter settings in real-time to match fluctuating signal environments.
- Intelligent Signal Classification: Filters can differentiate between noise, interference, and valuable data, optimizing the processing accordingly.
- Real-Time Corrections: AI models predict and correct distortions before they affect the signal integrity.
- Enhanced Efficiency: AI filters can reduce power consumption by optimizing resource allocation based on current signal conditions.
How the AI-Driven OTA Filter Works
- Signal Detection: The system continuously monitors incoming signals from various sources.
- Data Classification: AI algorithms classify the data into relevant categories, distinguishing between noise and useful signal.
- Adaptive Filtering: Based on the classification, the AI adjusts the filtering parameters to optimize signal clarity.
- Ongoing Refinement: The system learns and adjusts based on the feedback from real-time signal changes.
AI-powered OTA filters offer a revolutionary approach to signal processing by combining machine learning with real-time data analysis, enhancing both efficiency and quality in complex communication systems.
Comparison of Traditional vs. AI-Optimized OTA Filters
Feature | Traditional Filters | AI-Optimized Filters |
---|---|---|
Signal Adaptation | Fixed | Dynamic |
Noise Cancellation | Standard | Intelligent |
Real-Time Adjustments | Limited | Continuous |
Power Efficiency | Higher Consumption | Optimized |
Practical Benefits of AI-Driven Synthesis in OTA Filters for Mobile Communication
The integration of AI-driven synthesis into Over-The-Air (OTA) filter technology significantly enhances mobile communication systems. By using machine learning and AI algorithms to design and optimize filters, this approach allows for better performance, reduced interference, and more efficient spectrum management. AI-powered filters can automatically adapt to varying environmental conditions, ensuring continuous communication quality even in dynamic radio environments. This results in an overall improvement in signal strength, clarity, and reliability across diverse network settings.
Moreover, AI synthesis provides an efficient means of tuning OTA filters, reducing the need for manual intervention and speeding up the deployment of new technologies. It also helps to address key issues such as interference from neighboring signals, which are especially problematic in dense urban environments. By predicting and mitigating potential sources of interference, AI-powered OTA filters ensure uninterrupted communication and optimize network resources.
Key Benefits of AI-Driven OTA Filters
- Enhanced Signal Quality: AI synthesis can refine OTA filters to improve signal reception by dynamically adjusting to changes in the network and environment.
- Real-time Adaptation: Filters powered by AI continuously monitor and adapt to changing conditions, ensuring minimal disruptions.
- Reduced Interference: Machine learning models predict interference patterns and modify filter characteristics to eliminate unwanted signals.
- Faster Deployment: AI models streamline the design and implementation of OTA filters, cutting down on the time needed for network upgrades.
Operational Efficiency
- Cost Reduction: By minimizing the need for manual adjustments, AI-powered filters reduce operational costs associated with traditional filter deployment and tuning.
- Resource Optimization: AI algorithms can efficiently allocate network resources, ensuring maximum performance even in high-density areas.
- Scalability: AI models allow for easy scalability, making it possible to deploy advanced filtering solutions across various network types, from urban to rural environments.
Comparison of Traditional and AI-Enhanced OTA Filters
Filter Type | Manual Filter | AI-Powered Filter |
---|---|---|
Performance | Limited adaptability to changing environments | Dynamic adjustments based on real-time data |
Deployment Time | Longer, requires manual calibration | Faster, AI automates adjustments and optimizations |
Cost | Higher, due to manual interventions | Lower, optimized for efficiency |
AI-enhanced OTA filters represent a significant leap forward in mobile communication, offering superior performance, adaptability, and operational efficiency compared to traditional methods.
How AI-Driven Synthesis OTA Filter Enhances Data Transmission Precision
AI-assisted synthesis of OTA filters plays a crucial role in optimizing the accuracy of data transmission in communication systems. By integrating machine learning algorithms into the design and operational processes of filters, these systems can dynamically adapt to varying conditions, reducing signal distortions and improving overall transmission quality. The AI-driven approach is particularly useful in environments where conventional filter designs struggle to maintain consistent performance.
Incorporating AI into OTA filter technologies allows for continuous learning from the data received, enabling real-time adjustments to the filter characteristics. This adaptability results in more precise and error-free data transfer, particularly in environments with interference or fluctuating signal strengths. The self-optimizing nature of AI algorithms contributes significantly to maintaining high data integrity during transmission.
Key Benefits of AI-Driven OTA Filters
- Improved Noise Rejection: AI algorithms help identify and reject noise patterns more effectively, leading to cleaner signals.
- Dynamic Adaptability: Real-time adjustments ensure that the filter remains optimal even in changing signal conditions.
- Reduced Error Rates: Enhanced filtering reduces the likelihood of transmission errors and data corruption.
How It Works
- Signal Analysis: The AI system continuously analyzes incoming signals to detect patterns and potential interference.
- Filter Optimization: Based on the analysis, the AI adjusts the filter settings for optimal signal clarity and data accuracy.
- Real-Time Learning: Machine learning algorithms allow the system to improve over time, learning from past data and adjusting for future transmissions.
AI-driven synthesis of OTA filters allows systems to adapt to the complex and ever-changing conditions of modern communication networks, ensuring minimal loss of data during transmission.
Performance Comparison
Technology | Noise Rejection Efficiency | Error Rate |
---|---|---|
Traditional Filters | Moderate | High |
AI-Enhanced OTA Filters | High | Low |
Comparing AI-Driven Synthesis OTA Filters with Conventional Filtering Techniques
In recent years, AI-enhanced operational transconductance amplifier (OTA) filters have garnered significant attention for their ability to adapt and optimize signal processing in real-time. Unlike traditional filtering approaches, which rely heavily on predefined mathematical models, AI synthesis filters leverage machine learning algorithms to continuously improve their performance. This adaptability allows AI-driven filters to handle complex, non-linear signals more effectively compared to conventional methods, which can struggle with unpredictable or highly dynamic inputs.
Traditional filters, such as Butterworth, Chebyshev, or elliptic filters, typically operate with fixed frequency responses and require precise design parameters. While these methods are well-suited for predictable applications, they can be limited in flexibility and may not perform optimally in all scenarios. On the other hand, AI synthesis filters are dynamic, adjusting themselves based on real-time feedback from the input signal, providing more versatile and robust filtering solutions for modern electronic systems.
Key Differences Between AI Synthesis OTA Filters and Traditional Filtering Methods
- Adaptability: AI-based filters can adjust their characteristics in real-time, making them more versatile in handling varying signal conditions.
- Precision: Traditional filters are designed with fixed parameters, which can result in less precise performance when conditions change. AI filters, however, learn optimal parameters over time.
- Complexity Handling: AI filters can effectively handle complex, non-linear signals, while traditional filters may require multiple stages or fail to meet performance standards under certain conditions.
Performance Comparison
Feature | AI Synthesis OTA Filter | Traditional Filtering Methods |
---|---|---|
Adaptability | High – adjusts to changing signal conditions | Low – requires manual recalibration or redesign |
Precision | Dynamic precision based on learning algorithms | Fixed precision, determined during design phase |
Complex Signal Handling | Excellent for non-linear and dynamic signals | Can struggle with non-linear or unpredictable inputs |
"AI synthesis filters offer a level of flexibility and adaptability that traditional filtering methods simply cannot match. This makes them ideal for modern signal processing applications, where signal conditions are often dynamic and unpredictable."
Integrating AI-Based OTA Filter into Existing Network Infrastructure
AI-driven Over-the-Air (OTA) filters have become increasingly crucial for enhancing the performance and security of modern network systems. Integrating such filters into an established network infrastructure involves addressing several technical challenges while ensuring minimal disruption to current operations. A seamless integration requires careful planning and execution, with a focus on compatibility, scalability, and data handling efficiency.
The implementation of AI-based OTA filters must align with the existing network architecture, taking into account both the hardware and software requirements. It is essential to evaluate the current network capabilities and ensure that they can support the additional computational load required by AI algorithms. This will ensure optimized filtering without compromising system performance.
Key Steps in Integration
- Assessment of Current Network Infrastructure: Evaluate the existing network architecture, including hardware capabilities, software protocols, and security measures.
- Customization of AI Filter: Tailor the AI-based OTA filter to the specific needs of the network, considering traffic patterns and security concerns.
- Testing and Optimization: Conduct extensive testing to ensure the filter operates efficiently within the network's parameters. This includes load testing and checking for latency issues.
- Monitoring and Maintenance: Implement a robust monitoring system to track filter performance and make adjustments as necessary.
Considerations for Compatibility
Successful integration of an AI-based OTA filter requires ensuring that the filter can interface smoothly with legacy systems, without requiring complete overhauls of existing hardware or network setups.
The integration process also demands careful consideration of data throughput and processing power. Networks with limited capacity may need additional hardware resources, such as edge devices or cloud-based processing, to handle the increased demand. Coordination between network administrators and AI system developers is key to managing these resources effectively.
Example Integration Setup
Component | Requirement |
---|---|
Network Routers | High-performance routers with AI processing capability |
Server Capacity | Dedicated servers for AI algorithm processing and data analysis |
Security Protocols | Ensure integration with existing security measures (firewalls, encryption) |
Edge Devices | Optional for real-time data processing closer to the source |
Cost-Benefit Analysis of Implementing AI Synthesis OTA Filters in Telecom Systems
Telecommunication networks are increasingly relying on advanced filtering technologies to ensure optimal signal processing and interference management. The use of AI-powered synthesis filters, especially in Over-The-Air (OTA) systems, is gaining attention due to its potential to improve network performance while reducing operational costs. However, before adopting these filters, a thorough evaluation of the costs versus benefits is essential to make informed decisions that align with both technical requirements and financial constraints.
The integration of AI-driven OTA filters offers significant advantages in terms of adaptability and efficiency. However, it also involves certain investment and maintenance costs that must be carefully assessed. Below is a breakdown of the key factors to consider in a cost-benefit analysis.
Benefits of AI Synthesis OTA Filters
- Improved Signal Processing: AI filters enhance the precision of signal processing, minimizing interference and improving overall quality.
- Cost Savings: Automation reduces the need for manual adjustments and reduces human error, cutting operational costs over time.
- Adaptability: These filters can quickly adjust to changes in network conditions, leading to more reliable performance in varying environments.
Costs to Consider
- Initial Investment: The development and deployment of AI filters require substantial initial capital for software and hardware integration.
- Training and Expertise: Telecom companies must invest in staff training or hire specialized personnel to manage the system.
- Maintenance and Upgrades: Ongoing updates to the AI models and regular maintenance are necessary to keep the system running optimally.
Summary Table
Factors | Benefits | Costs |
---|---|---|
Signal Processing | Enhanced quality and reduced interference | None |
Cost Savings | Lower operational costs over time | Initial investment for integration |
Adaptability | Higher network reliability | Need for continuous training and upgrades |
Important Consideration: While AI filters can significantly improve network performance, their effectiveness depends on the ongoing investment in technology upgrades and skilled personnel. Companies must balance long-term savings with the costs of integration and maintenance.