Ai for Radiology
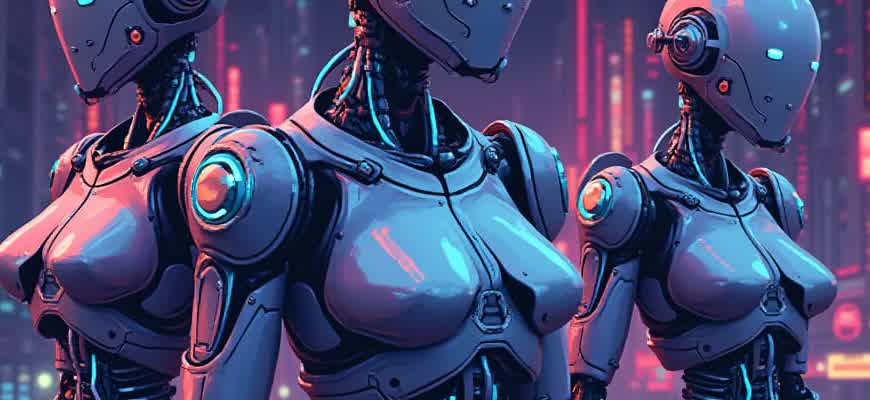
Artificial intelligence (AI) is revolutionizing the field of radiology, enhancing diagnostic capabilities and improving patient outcomes. AI algorithms, particularly those based on deep learning, are increasingly being integrated into radiological workflows to assist with the interpretation of medical images. This advancement supports radiologists in identifying anomalies more accurately and efficiently, reducing the time required for diagnoses and minimizing human error.
AI’s primary applications in radiology include:
- Automated detection of diseases, such as tumors, fractures, and neurological disorders.
- Image enhancement, improving the clarity and quality of scans.
- Predictive analytics to assess disease progression and potential risks.
Key Insight: AI has the potential to significantly reduce the radiologist's workload while maintaining or even improving diagnostic accuracy, especially in routine or complex cases.
One of the most critical aspects of AI in radiology is its ability to process and analyze large datasets quickly. This capability allows AI systems to provide more accurate and consistent results than traditional manual interpretation, which can be influenced by fatigue or subjective judgment.
Incorporating AI tools into the clinical environment involves addressing several key factors:
- Integration with existing healthcare systems and imaging devices.
- Ensuring data security and patient privacy.
- Training and educating radiologists on AI-assisted workflows.
AI Application | Potential Benefit |
---|---|
Automated Detection | Faster diagnosis, reduced human error |
Image Enhancement | Improved scan quality, clearer images |
Predictive Analytics | Earlier detection of disease, better risk management |
AI for Radiology: Transforming Diagnostic Practices
The integration of artificial intelligence (AI) into radiology is revolutionizing diagnostic workflows, enhancing both the speed and accuracy of image interpretation. AI algorithms can process vast amounts of imaging data in a fraction of the time it takes a human radiologist, thereby enabling faster diagnosis and reducing workload. These systems can also identify patterns that may be too subtle for the human eye, improving detection of conditions such as cancers, fractures, and neurological diseases.
As AI technology continues to evolve, its impact on radiology practice becomes increasingly profound. The adoption of AI tools not only enhances clinical decision-making but also provides opportunities for personalized patient care. Radiologists are empowered to focus on complex cases while AI handles routine image analysis, allowing for more efficient resource utilization in medical settings.
Key Benefits of AI in Radiology
- Increased Accuracy: AI systems can identify complex patterns in medical images that may be missed by human radiologists.
- Faster Diagnoses: AI can quickly analyze large volumes of imaging data, reducing time to diagnosis.
- Reduced Workload: Automation of routine tasks allows radiologists to focus on more complex cases.
Applications of AI in Radiology
- Automated Image Interpretation: AI algorithms can assist in detecting abnormalities such as tumors, hemorrhages, or fractures in radiographic images.
- Predictive Analytics: AI models can predict patient outcomes based on imaging data, assisting in treatment planning and follow-up care.
- Radiomics: AI is used to extract and analyze quantitative data from images, offering insights into tissue characteristics and disease progression.
AI-driven technologies are not just assistants in diagnosis; they are becoming integral partners in providing high-quality, efficient, and personalized care to patients.
Challenges and Considerations
Challenge | Consideration |
---|---|
Data Quality | The accuracy of AI models depends on the quality of the training data used. Insufficient or biased data can lead to inaccurate predictions. |
Integration with Existing Systems | AI tools must seamlessly integrate with current radiology workflows and medical imaging systems for maximum efficiency. |
Ethical and Legal Concerns | Ensuring patient privacy, security of data, and clarity on AI’s role in decision-making are key considerations. |
How AI Enhances Speed in Radiological Image Interpretation
The application of artificial intelligence (AI) in radiology has proven to significantly accelerate the process of image analysis. By using machine learning algorithms, AI systems can instantly analyze vast amounts of imaging data, allowing radiologists to focus more on critical cases and treatment planning rather than spending excessive time on routine image interpretation. With the ability to process images rapidly, AI helps to detect and highlight abnormalities in real-time, reducing delays in diagnosis.
Furthermore, AI models are capable of learning from large datasets, continuously improving their ability to identify patterns, anomalies, and subtle changes in medical images. This leads to an overall enhancement in diagnostic accuracy and speed. In many cases, AI can provide initial interpretations, flagging areas of concern for further review, which speeds up workflow without compromising diagnostic quality.
Key Factors Contributing to Speed Enhancement
- Automation of Routine Tasks: AI handles repetitive tasks like segmenting organs or tissues in images, allowing radiologists to focus on more complex diagnostic decisions.
- Real-Time Analysis: With machine learning algorithms, AI can quickly process high volumes of data, providing instant results that help accelerate decision-making.
- Integration with Existing Systems: AI tools seamlessly integrate with Picture Archiving and Communication Systems (PACS), enabling faster retrieval and analysis of images.
Examples of AI-Driven Speed Improvements in Radiology
- Chest X-Ray Interpretation: AI has been shown to reduce the time for analyzing chest X-rays by automating the detection of lung diseases like pneumonia or tuberculosis.
- CT Scan Review: AI algorithms can detect brain hemorrhages or fractures in CT scans much faster than manual review, significantly shortening the time from image acquisition to diagnosis.
- Breast Cancer Screening: AI-powered tools help in processing mammograms quickly, detecting potential signs of cancer at an early stage.
Impact on Radiology Workflow
"By reducing the time spent on routine tasks, AI empowers radiologists to focus on the clinical aspects of patient care, ultimately improving both efficiency and the quality of patient outcomes."
AI's rapid processing ability has a direct impact on the overall radiology workflow. Tasks that traditionally took a considerable amount of time are now completed much faster, reducing turnaround times and increasing the overall capacity of radiology departments. This leads to more timely diagnoses, which can be crucial for patient outcomes.
Comparing Traditional vs AI-Enhanced Analysis Speed
Task | Traditional Time (mins) | AI Time (mins) |
---|---|---|
Chest X-Ray Analysis | 10-15 | 2-5 |
CT Brain Scan Review | 20-30 | 5-10 |
Mammogram Interpretation | 15-20 | 3-6 |
Integrating Artificial Intelligence with Radiology Systems
Integrating AI technologies into existing radiology systems is a significant step toward enhancing diagnostic accuracy and workflow efficiency. The key challenge lies in seamlessly merging advanced AI capabilities with legacy software platforms commonly used in medical imaging, ensuring minimal disruption while maximizing benefits. AI can assist radiologists in detecting patterns in medical images that may be difficult to identify manually, improving both the speed and precision of diagnoses. However, successful integration requires a careful approach that takes into account system compatibility, data privacy concerns, and user adoption.
Several factors influence the smooth incorporation of AI into radiology software, ranging from technical aspects like interoperability to practical considerations such as user interface design. Integration must also involve the support of robust data pipelines, as AI models require access to high-quality imaging data to function properly. The collaboration between AI developers and radiology software vendors is crucial to create systems that are both intuitive and scalable.
Key Considerations for AI Integration
- Interoperability: Ensure that AI solutions can work with a variety of existing imaging modalities and software platforms without the need for extensive modifications.
- Data Privacy: Adhere to legal and ethical guidelines such as HIPAA to protect patient data during the integration process.
- Real-Time Processing: The AI tools should be able to analyze images in real-time or near real-time without causing delays in the diagnostic process.
Integration Challenges
Effective AI integration requires overcoming the challenge of adapting AI models to the specific data formats and protocols used by legacy radiology software systems.
- Compatibility with legacy hardware and software
- Training AI models on high-quality, diverse imaging data
- Ensuring the continuous updating of AI algorithms for improved performance
Example of AI Integration in Radiology Software
AI Feature | Software Integration Aspect |
---|---|
Automated Tumor Detection | Integration with PACS systems for seamless image review |
Image Segmentation | Alignment with DICOM standards for image processing |
Decision Support | Embedding AI results within radiologist's workflow interface |
Training Radiologists to Work with AI-Driven Tools
As AI continues to transform the field of radiology, it is essential for radiologists to be proficient not only in interpreting medical images but also in collaborating with AI-driven tools. The integration of these technologies demands a shift in the educational approach, blending traditional radiology training with an understanding of AI's capabilities and limitations. This dual expertise will enable radiologists to enhance their diagnostic accuracy while making informed decisions about the application of AI in clinical practice.
Effective training programs must ensure that radiologists are comfortable using AI tools and interpreting their outputs. This involves not only understanding the mechanics of AI models but also recognizing when human intervention is needed. Given the rapid evolution of AI technologies, continuous education is crucial to maintain expertise and adapt to new advancements.
Key Areas of Focus in AI Training for Radiologists
- Understanding AI Model Fundamentals: Radiologists should learn about the basic principles of machine learning, neural networks, and how these models are applied in medical imaging.
- Critical Evaluation of AI Output: Training should include how to interpret AI-generated results, including the recognition of false positives or false negatives and understanding the reasoning behind the AI's conclusions.
- Integration into Clinical Workflow: Radiologists must be trained in how to incorporate AI tools into their daily practice without disrupting established workflows.
Training Methods
- Interactive Workshops: Hands-on sessions where radiologists work directly with AI tools to understand their functionality and limitations.
- Simulations and Case Studies: Scenarios that replicate real-world conditions, allowing radiologists to practice making decisions with AI assistance in a safe environment.
- Continuous Professional Development: Online courses, webinars, and conferences dedicated to the latest advancements in AI for radiology.
"AI tools should be viewed as an extension of a radiologist's skill set, not a replacement for human expertise."
Training Metrics and Success Indicators
Metric | Success Indicator |
---|---|
Knowledge Retention | Radiologists demonstrate a clear understanding of AI models and their applications in real-world scenarios. |
Clinical Outcomes | Improved diagnostic accuracy and faster decision-making with the use of AI tools. |
Adaptation to Workflow | Efficient integration of AI into routine practices without causing workflow disruptions. |
Reducing Human Error in Medical Imaging through AI
Medical imaging is a critical component in diagnosing a variety of conditions, but human error remains a significant concern in the interpretation of these images. Radiologists are tasked with reviewing complex images under time pressure, which can lead to oversights and misinterpretations. Artificial intelligence has emerged as a powerful tool to assist in minimizing these errors and improving the overall accuracy of diagnoses.
AI systems, through machine learning and deep learning algorithms, can assist radiologists by automating certain aspects of the imaging review process. By analyzing vast amounts of data, AI can identify patterns and anomalies that might be overlooked by a human, thereby enhancing the speed and reliability of diagnoses.
Key Benefits of AI in Reducing Human Error
- Automated Anomaly Detection: AI models can quickly highlight potential issues in medical images, allowing radiologists to focus on areas that require deeper analysis.
- Consistency and Accuracy: AI systems offer consistent interpretation of images, reducing the chances of misdiagnosis due to fatigue or varying human judgment.
- Speed and Efficiency: AI can process and analyze images faster than humans, accelerating the diagnostic workflow and enabling earlier treatment decisions.
How AI Reduces Errors in Medical Imaging
- Enhancing Image Quality: AI algorithms can be used to enhance the quality of medical images, improving visibility of details that might be missed in lower-quality scans.
- Pattern Recognition: Machine learning models are capable of learning from vast datasets, enabling them to recognize rare conditions and subtle abnormalities with high precision.
- Reduction of Fatigue-Related Errors: Radiologists often face long working hours, leading to mental fatigue. AI systems can handle the initial image screening, allowing doctors to focus on more complex cases.
"The integration of AI into radiology is not about replacing human expertise but enhancing it to achieve a higher standard of care with fewer mistakes."
Comparison of Human and AI Error Rates in Radiology
Factor | Human Error Rate | AI Error Rate |
---|---|---|
Missed Diagnoses | 15-20% | 5-10% |
False Positives | 10-15% | 3-5% |
Overall Diagnostic Accuracy | 85-90% | 90-95% |
Enhancing Early Disease Detection with AI Algorithms
Artificial intelligence (AI) has significantly transformed early disease diagnosis in radiology. With the advent of machine learning and deep learning models, the ability to detect abnormalities in medical images has improved, enabling clinicians to identify health issues at much earlier stages. AI-driven algorithms can analyze large volumes of radiological data quickly and with high accuracy, often identifying subtle signs that may be missed by the human eye.
AI-powered tools in radiology are particularly useful in detecting diseases such as cancer, cardiovascular conditions, and neurological disorders. By leveraging advanced image recognition techniques, these algorithms help radiologists to enhance their diagnostic precision and reduce the risk of human error. This leads to better outcomes for patients, as earlier detection generally correlates with more effective treatment options.
Key Benefits of AI in Early Disease Detection
- Speed and Efficiency: AI can process and analyze medical images significantly faster than human radiologists, enabling quicker diagnosis and treatment.
- Increased Accuracy: AI algorithms can detect patterns in imaging data that are often invisible to the naked eye, increasing diagnostic accuracy.
- Consistency: Unlike human radiologists, AI systems do not suffer from fatigue or variability, ensuring consistent performance over time.
Applications of AI in Radiology for Early Disease Detection
- Cancer Screening: AI algorithms are frequently used for identifying early-stage cancers, such as lung and breast cancer, through CT scans and mammograms.
- Cardiovascular Conditions: AI tools can analyze heart imaging data, identifying early signs of heart disease or strokes before major events occur.
- Neurological Disorders: AI can assist in the early detection of conditions like Alzheimer's and multiple sclerosis by analyzing brain scans.
"AI systems not only improve early detection but also provide decision support, offering radiologists valuable insights to complement their expertise."
Example: AI in Breast Cancer Detection
Algorithm Type | Detection Rate | Impact |
---|---|---|
Deep Learning Networks | 95% accuracy | Improved early-stage detection by identifying microcalcifications and abnormal masses |
Convolutional Neural Networks | 90% accuracy | Reduced false positives and unnecessary biopsies |
Evaluating the Cost-Effectiveness of AI Adoption in Radiology Departments
Incorporating artificial intelligence into radiology departments has the potential to transform clinical workflows, improve diagnostic accuracy, and reduce operational costs. However, the decision to implement AI tools requires a thorough analysis of both the benefits and costs involved. This includes upfront investments in technology, integration with existing systems, training of medical professionals, and potential savings through enhanced efficiency. A proper cost-benefit assessment is crucial for determining the long-term value of AI in radiology departments.
On the cost side, the initial expenditure on AI tools, along with ongoing maintenance and support, can be significant. Additionally, there are hidden costs such as staff training, system integration, and potential disruptions to existing workflows during the transition period. Despite these challenges, AI technologies can provide a return on investment by reducing the workload on radiologists, decreasing the number of diagnostic errors, and speeding up the time for image analysis. These advantages may lead to higher throughput and better patient outcomes, making the initial investment worthwhile.
Cost Factors to Consider
- Initial Setup Costs: Includes purchasing software, hardware, and setting up the AI infrastructure.
- Ongoing Maintenance: Regular software updates, system support, and troubleshooting costs.
- Staff Training: Time and resources dedicated to training radiologists and other medical staff on the new system.
- Integration with Existing Systems: Costs related to adapting AI to existing imaging and medical records systems.
Potential Benefits of AI in Radiology
- Improved Diagnostic Accuracy: AI algorithms can assist radiologists in identifying patterns and anomalies, potentially increasing diagnostic precision.
- Increased Efficiency: AI tools can process large volumes of images more quickly, allowing radiologists to focus on complex cases.
- Cost Savings in the Long Term: By automating repetitive tasks and reducing diagnostic errors, AI can lead to significant reductions in the cost of misdiagnoses and patient rework.
- Enhanced Patient Outcomes: Faster diagnosis and more accurate imaging can lead to better treatment outcomes for patients.
Cost-Benefit Comparison
Factor | Estimated Cost | Expected Benefit |
---|---|---|
Initial Setup | $500,000 - $1,000,000 | Faster image processing, reduced diagnostic errors |
Training and Integration | $100,000 | Increased staff competency, better workflow integration |
Ongoing Maintenance | $50,000/year | Improved accuracy, long-term system reliability |
Important Consideration: While AI adoption may require significant initial investment, the long-term benefits in terms of operational efficiency, accuracy, and patient care may outweigh these costs.
Overcoming Data Privacy and Security Concerns in AI-Driven Radiology
Data privacy and security issues remain one of the most critical challenges in implementing artificial intelligence (AI) in radiology. The healthcare industry, especially radiology, handles vast amounts of sensitive personal data, including medical imaging and patient histories. With the increasing use of AI to assist in diagnostics, ensuring the protection of this data is essential to maintain patient trust and comply with regulatory standards. Moreover, AI models trained on medical data must adhere to stringent privacy regulations such as HIPAA in the U.S. or GDPR in Europe, which govern the handling and storage of patient data.
Efforts to address these challenges include a combination of technological solutions, regulatory frameworks, and best practices in data management. Protecting patient privacy while leveraging the capabilities of AI requires balancing data accessibility with strong security measures. To achieve this, AI developers and healthcare providers must work together to ensure that data is encrypted, anonymized, and securely stored. Additionally, employing methods like federated learning can help mitigate privacy risks while still enabling AI models to learn from decentralized medical data.
Key Approaches to Ensuring Data Privacy and Security
- Data Encryption: All patient data, including medical images, should be encrypted both in transit and at rest to protect it from unauthorized access.
- Anonymization and Pseudonymization: Removing personally identifiable information (PII) from datasets helps to safeguard privacy while still allowing AI algorithms to function effectively.
- Federated Learning: This approach enables AI models to be trained across multiple healthcare institutions without sharing the actual patient data, ensuring that sensitive information is kept within each institution's secure environment.
Regulatory Compliance
AI-driven radiology solutions must be designed to comply with both local and international privacy regulations. Compliance with laws such as HIPAA or GDPR is essential for maintaining data security and avoiding legal ramifications.
Regulation | Region | Key Requirements |
---|---|---|
HIPAA | United States | Data encryption, access control, and breach notifications |
GDPR | European Union | Consent-based data collection, right to erasure, and data portability |
"The application of AI in radiology should never compromise the confidentiality and integrity of patient data. Strict adherence to regulatory standards ensures that AI technologies in healthcare can advance while maintaining privacy protections."