Ai X-ray
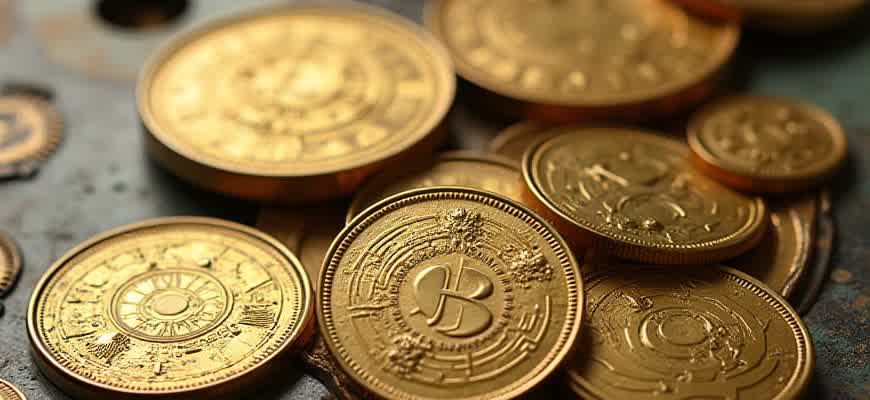
The integration of artificial intelligence (AI) in medical imaging, particularly in X-ray analysis, has significantly transformed diagnostic practices. By leveraging advanced algorithms, AI can detect patterns and anomalies in X-ray images with exceptional accuracy, often surpassing human capabilities in speed and precision.
AI applications in X-ray technology have become increasingly vital in detecting a range of conditions, from fractures to lung diseases. Key benefits include:
- Faster image processing times
- Improved diagnostic accuracy
- Automated detection of abnormalities
- Reduction in human error and fatigue
"AI technologies are revolutionizing the way we interpret X-rays, enabling faster and more reliable diagnoses."
For a more comprehensive view, AI-based systems analyze X-ray images in various ways. These include:
- Enhancement of image quality for better visibility of issues
- Automated reporting systems to generate immediate results for healthcare professionals
- Integration with patient data to track medical history and improve overall diagnostic accuracy
Application | Benefit |
---|---|
Fracture Detection | AI can automatically identify bone fractures, minimizing the risk of missed diagnoses. |
Lung Disease Detection | AI models can detect early signs of conditions like pneumonia or cancer from chest X-rays. |
AI in X-Ray: Revolutionizing Diagnostic Imaging
Artificial intelligence (AI) has significantly transformed the landscape of medical imaging, particularly in the realm of X-ray diagnostics. AI algorithms, powered by deep learning, have the ability to analyze complex X-ray images with unprecedented speed and accuracy. This has opened up new possibilities for early detection, personalized treatment planning, and reducing the workload of radiologists. By leveraging AI tools, clinicians are now able to detect abnormalities that may have been missed by the human eye, increasing both efficiency and patient safety.
The integration of AI into X-ray diagnostics is not just about improving detection capabilities. It is also about optimizing the workflow in medical facilities, providing real-time analysis, and aiding radiologists with decision support. AI models are trained on vast datasets of X-ray images, allowing them to identify patterns and anomalies quickly, leading to faster diagnoses and better patient outcomes.
How AI Enhances X-Ray Imaging
AI-based tools are revolutionizing how radiologists interpret X-ray images. The main advantages include:
- Faster Diagnosis: AI can process images quickly, providing results in seconds, which can be critical in emergency situations.
- Higher Accuracy: With advanced pattern recognition, AI can identify subtle anomalies that human eyes might miss, improving diagnostic precision.
- Reduced Human Error: AI minimizes the potential for human error, offering consistent results regardless of fatigue or workload.
Key Applications of AI in X-Ray Imaging
AI is applied in several areas of X-ray diagnostics, providing support in identifying and analyzing conditions such as:
- Pneumonia: AI can help detect early signs of pneumonia from chest X-rays, allowing for faster treatment.
- Fractures and Bone Diseases: Machine learning algorithms analyze bone X-rays to detect fractures, tumors, or signs of osteoporosis.
- Lung Cancer: AI models are used to identify nodules or other suspicious patterns in lung X-rays, aiding in early cancer detection.
Comparison: Traditional vs. AI-Enhanced X-Ray Diagnostics
Feature | Traditional X-Ray | AI-Enhanced X-Ray |
---|---|---|
Speed | Time-consuming, manual interpretation | Instant results, real-time analysis |
Accuracy | Susceptible to human error, missed abnormalities | High precision with reduced risk of oversight |
Workload | Heavy workload for radiologists | AI assists, reducing radiologist burden |
"AI is not just a tool for automating tasks; it's a partner in improving the quality of care for patients by enhancing diagnostic accuracy and providing faster results." - Radiology Expert
How AI X-ray Enhances Image Clarity and Detail
AI-driven X-ray technologies have revolutionized medical imaging by significantly improving the clarity and resolution of images. Traditional X-ray systems sometimes struggle with subtle or overlapping details, especially in dense tissue areas. AI algorithms, leveraging deep learning models, enhance image quality by identifying features that may be difficult for human eyes to detect, thus enabling more accurate diagnoses.
Through the integration of machine learning, AI enhances the overall diagnostic process by processing raw data into sharper, more defined visual representations. This provides clearer differentiation between tissues, fractures, and foreign objects, all while minimizing exposure to radiation.
Key Improvements with AI in X-ray Imaging
- Improved resolution: AI optimizes pixel-level detail, enhancing fine structures like bones or soft tissues.
- Noise reduction: The AI filters out irrelevant noise from images, improving clarity in low-contrast areas.
- Faster processing: AI reduces the time required to generate high-quality images, speeding up diagnosis and treatment planning.
- Artifact correction: AI can correct distortions or artifacts that may appear due to patient movement or equipment limitations.
AI X-ray Workflow
- Image Acquisition: The X-ray machine captures the initial image of the patient.
- AI Processing: The captured image is then processed using AI algorithms to enhance details and correct errors.
- Enhanced Output: The processed image is presented to the radiologist with improved clarity and diagnostic features.
"AI X-ray technology not only enhances image quality but also supports faster, more accurate decision-making by medical professionals."
Comparison Table: Traditional vs. AI-Enhanced X-ray
Feature | Traditional X-ray | AI-Enhanced X-ray |
---|---|---|
Image Resolution | Standard resolution with limitations in dense areas | High resolution with improved clarity, especially in complex regions |
Noise and Artifacts | Potential for visible noise and artifacts | Noise reduction and artifact correction |
Processing Time | Longer processing time for detailed analysis | Faster image processing with detailed results |
Integration of AI in Radiology Systems
As artificial intelligence continues to advance, its integration into medical imaging technologies, such as X-ray diagnostics, promises to enhance healthcare systems worldwide. AI algorithms can assist radiologists by improving diagnostic accuracy, speed, and workflow efficiency. These technologies are designed to analyze medical images more precisely than traditional methods, thus supporting clinicians in detecting and diagnosing conditions with greater certainty.
However, the integration of AI-powered X-ray systems into existing healthcare infrastructure is not without challenges. Adapting to these new technologies requires significant changes in hospital operations, system compatibility, and data management. Ensuring that AI tools are compatible with current medical software is critical for smooth implementation.
Key Considerations for Integration
- System Compatibility: AI X-ray solutions must integrate seamlessly with existing Picture Archiving and Communication Systems (PACS) and Radiology Information Systems (RIS).
- Training and Education: Radiologists and healthcare professionals need training to effectively use AI-driven tools and interpret the results generated by AI systems.
- Data Security and Privacy: Implementing AI technologies requires robust data protection measures to safeguard patient information in compliance with healthcare regulations, such as HIPAA.
Steps to Implement AI X-ray Systems
- Evaluation of Technology Providers: Selecting a reliable AI technology partner is crucial for ensuring a smooth integration process.
- Pilot Testing: A phased approach, starting with pilot testing, helps to identify potential issues before full implementation.
- Continuous Monitoring: After implementation, ongoing monitoring and updates are necessary to address performance issues and improve the system's capabilities.
Benefits of AI X-ray Integration
Benefit | Description |
---|---|
Improved Accuracy | AI algorithms can detect subtle patterns that human radiologists may miss, increasing diagnostic precision. |
Faster Diagnosis | AI can process images and generate results quickly, reducing wait times for patients and allowing radiologists to focus on complex cases. |
Operational Efficiency | Automating routine tasks and reducing workload enables healthcare providers to allocate resources more effectively. |
AI integration into radiology systems is not just a technological upgrade; it’s a significant shift in how medical professionals interact with diagnostic tools, potentially transforming healthcare delivery.
Reducing Radiation Exposure with AI-Powered X-rays
AI-driven advancements in radiology are significantly improving the safety of X-ray imaging by minimizing the amount of radiation required to obtain high-quality images. Traditional X-rays often involve relatively high doses of radiation, which can increase the risk of long-term health issues. However, artificial intelligence is now being used to optimize X-ray protocols, ensuring the necessary image clarity while reducing exposure to harmful radiation.
The integration of AI algorithms into imaging systems helps adjust exposure settings automatically based on patient characteristics, such as body size, and the specific clinical purpose of the scan. This ability to tailor radiation levels in real-time contributes to a safer experience for patients, particularly those requiring frequent imaging, such as cancer patients or children.
Key Benefits of AI in Reducing Radiation
- Precision in Exposure Control: AI systems can calculate the optimal radiation dose based on real-time data, ensuring that patients receive the minimum necessary exposure for accurate imaging results.
- Enhanced Image Quality: Even with lower radiation doses, AI can enhance image quality, improving diagnostic accuracy without compromising patient safety.
- Adaptive Learning: AI algorithms continuously learn from new imaging data, improving their ability to make decisions and further reducing unnecessary radiation in future scans.
How AI Adjusts Radiation Levels
- Automatic Dose Adjustment: AI-powered systems detect variables like patient size, age, and body composition to determine the most effective radiation settings.
- Real-Time Image Enhancement: Algorithms process and enhance low-dose images, compensating for any reduction in clarity while preserving diagnostic value.
- Continuous Monitoring: AI monitors the performance of imaging systems, ensuring radiation doses remain within safe thresholds, especially in high-risk patients.
"AI technologies are not just about improving the efficiency of medical imaging; they are crucial in protecting patients from unnecessary radiation exposure while maintaining diagnostic accuracy."
Comparison of Traditional vs. AI-Powered X-ray Systems
Feature | Traditional X-ray | AI-Powered X-ray |
---|---|---|
Radiation Dose | Higher dose required | Optimized dose, lower exposure |
Image Quality | Dependent on manual settings | Enhanced with AI algorithms |
Real-Time Adaptability | Limited adjustment | Automatic adjustments based on patient and scan conditions |
Enhancing Diagnostic Speed with AI X-ray Solutions
Artificial Intelligence (AI) has made significant strides in medical imaging, particularly in the realm of X-ray diagnostics. Traditional methods rely heavily on radiologists' expertise to identify and interpret abnormalities, but AI technologies now offer the potential to revolutionize the speed and accuracy of this process. By leveraging machine learning models trained on vast datasets, AI systems can assist in detecting and diagnosing conditions much faster than human counterparts alone. This ability not only shortens waiting times for patients but also enables medical professionals to focus their attention on the most critical cases.
AI-powered X-ray solutions process images quickly, reducing the time required to make diagnoses. Through advanced algorithms, these tools can identify patterns and anomalies with remarkable precision, significantly cutting down the diagnostic timeline. This has a profound impact on emergency care settings, where every second matters in providing the right treatment for patients in critical conditions.
Key Advantages of AI in X-ray Diagnostics
- Faster Diagnosis: AI algorithms analyze X-ray images in a fraction of the time it would take a human radiologist, facilitating quicker decision-making.
- Increased Accuracy: By learning from vast amounts of medical data, AI systems can identify subtle abnormalities that might be overlooked by the human eye.
- Enhanced Workflow Efficiency: Radiologists can prioritize urgent cases, as AI systems filter out routine or less critical diagnoses.
Steps AI Uses to Accelerate Diagnosis
- Image Preprocessing: AI systems first preprocess the X-ray image to enhance its clarity and highlight key features.
- Pattern Recognition: Using trained models, AI detects various conditions such as fractures, tumors, or infections based on image patterns.
- Automated Reporting: After processing, AI generates reports that outline findings, enabling faster clinical decision-making.
"AI X-ray technology doesn’t replace the role of radiologists but significantly augments their ability to make faster and more accurate diagnoses, ultimately improving patient outcomes."
Comparison of Traditional vs AI-Enhanced X-ray Diagnosis
Feature | Traditional X-ray | AI-Powered X-ray |
---|---|---|
Diagnosis Speed | Hours or days depending on case load | Minutes to hours depending on complexity |
Accuracy | Dependent on radiologist experience | High accuracy, with machine learning validation |
Workflow | Manual interpretation, often with a backlog | Automated analysis, prioritization of urgent cases |
AI X-ray for Faster Triage in Emergency Settings
In emergency healthcare environments, rapid decision-making is essential to prioritize patients based on the severity of their conditions. Traditional methods of assessing injuries, particularly using X-ray imaging, can be time-consuming and dependent on human interpretation. AI-driven X-ray analysis systems can significantly expedite this process by quickly assessing images and providing immediate suggestions for prioritization, improving overall triage efficiency.
Artificial intelligence, particularly deep learning models, is transforming how X-ray images are processed. These models can rapidly identify fractures, internal bleeding, or other critical issues, offering real-time results that can assist medical professionals in determining the most urgent cases. This technology reduces the time required for manual evaluation, allowing medical staff to focus on critical interventions rather than diagnostic delays.
Key Advantages of AI X-ray in Emergency Triage
- Speed: AI algorithms can process X-ray images within seconds, enabling faster identification of life-threatening conditions.
- Accuracy: AI systems trained on large datasets often achieve higher accuracy than human interpretation alone, reducing the likelihood of missed diagnoses.
- Prioritization: AI can flag severe cases and suggest a triage protocol, helping medical teams make quicker decisions on patient care.
Through faster triage, emergency departments can direct their resources more effectively, ensuring that patients with the most urgent needs receive prompt attention. This innovation not only enhances patient outcomes but also alleviates the burden on healthcare professionals in high-pressure environments.
Considerations for Implementation
- Integration with Existing Systems: AI X-ray tools must seamlessly integrate with hospital infrastructure, such as electronic medical records and existing diagnostic equipment.
- Training and Validation: Ongoing training of AI models is necessary to maintain accuracy, particularly as new medical conditions and treatment methods emerge.
- Ethical Concerns: Ensuring that AI-based systems are used responsibly, with safeguards in place to protect patient data and decision-making autonomy.
"AI has the potential to revolutionize emergency medicine by enhancing triage speed and accuracy, ultimately saving lives in critical situations."
Benefit | Impact |
---|---|
Reduced Wait Times | Faster processing of X-ray images leads to quicker diagnosis and treatment. |
Improved Diagnostic Precision | AI can detect anomalies that might be missed by human eyes, ensuring fewer misdiagnoses. |
Resource Efficiency | Healthcare staff can focus on the most critical patients, improving resource allocation. |
Cost Savings in Healthcare with AI X-ray Solutions
Artificial intelligence has made significant strides in the healthcare sector, especially in the field of medical imaging. By integrating AI-powered X-ray systems, healthcare facilities can reduce operational costs while improving diagnostic accuracy. AI algorithms can quickly analyze X-ray images, reducing the time required for human interpretation. This not only accelerates decision-making but also minimizes errors, leading to cost-effective care delivery.
AI-driven solutions provide multiple cost-saving opportunities across various levels of healthcare operations. From reduced need for additional diagnostic tests to more efficient use of staff, AI X-ray systems offer a substantial return on investment. Below are key ways in which AI in X-ray technology contributes to financial savings for healthcare providers.
Key Cost-Saving Benefits of AI X-ray Solutions
- Improved Diagnostic Accuracy: Reducing misdiagnoses leads to fewer unnecessary treatments and hospital stays, which directly lowers healthcare expenses.
- Reduced Labor Costs: AI reduces the workload of radiologists by automating routine image analysis, allowing them to focus on more complex cases.
- Enhanced Workflow Efficiency: Faster image interpretation speeds up patient throughput, leading to greater productivity and less time spent per patient.
- Lower Equipment Costs: AI systems can optimize the use of existing imaging equipment, ensuring better utilization and extending the life of medical devices.
"AI X-ray technology has the potential to revolutionize cost management in healthcare, delivering both financial and clinical benefits."
Cost-Saving Potential of AI X-ray Solutions
- Fewer unnecessary follow-up procedures due to accurate and reliable results from AI-based imaging.
- Decreased operational costs through the automation of repetitive tasks, reducing the need for additional staff and cutting overhead expenses.
- Better resource allocation through real-time image analysis, improving hospital and clinic efficiency.
Financial Impact of AI in Medical Imaging
Benefit | Impact |
---|---|
Reduced Diagnostic Errors | Lower medical error-related costs and legal fees. |
Faster Image Analysis | Increased patient throughput and optimized workforce utilization. |
Operational Efficiency | Lower labor and operational costs through automation. |
Future Prospects: Scaling AI X-ray for Global Healthcare Needs
Artificial intelligence (AI) technologies are revolutionizing healthcare by enabling advanced diagnostic tools that are more accurate, efficient, and scalable. Among the most promising innovations is AI-driven X-ray imaging, which has the potential to significantly improve medical diagnostics worldwide. As AI algorithms continue to evolve, their integration into healthcare systems presents an opportunity to address the global shortage of medical professionals and imaging equipment, especially in underserved areas. The ability of AI to interpret X-ray images faster and more accurately than traditional methods could vastly improve early detection rates for diseases such as tuberculosis, pneumonia, and cancer.
Scaling AI-powered X-ray systems presents both opportunities and challenges. While AI has the potential to reduce diagnostic errors and improve patient outcomes, its widespread adoption requires significant infrastructure development, training, and regulatory compliance. Ensuring that AI X-ray technology is accessible and affordable across various healthcare settings–particularly in low-resource regions–will be key to its global impact. In addition, ongoing advancements in AI model robustness and interpretability are essential to build trust and reliability in these systems among healthcare professionals and patients alike.
Key Areas for Scaling AI X-ray Technology
- Infrastructure Development: Expanding healthcare infrastructure, particularly in rural or low-income regions, is critical to ensure that AI X-ray systems can be deployed and effectively utilized. This includes both physical facilities and digital networks capable of supporting AI-driven solutions.
- Training and Education: Healthcare workers must be trained to use AI-powered diagnostic tools efficiently. Continuous professional development and educational programs are essential to ensure proper implementation and maximize the benefits of AI-assisted diagnostics.
- Cost Reduction: The affordability of AI X-ray systems will determine their widespread adoption. Efforts to reduce manufacturing and operational costs, along with increased competition, will help make these technologies more accessible worldwide.
Challenges to Overcome
- Data Privacy and Security: Ensuring that patient data is protected and handled securely when using AI-driven diagnostics is critical to maintaining trust in these technologies.
- Regulatory Hurdles: Different countries have varying standards and regulations for medical technologies. Streamlining the regulatory approval process for AI X-ray systems is crucial for faster deployment on a global scale.
- Integration into Existing Systems: The integration of AI-powered X-ray technologies with existing healthcare infrastructure, including electronic health records (EHR) and diagnostic platforms, is a complex but necessary step for effective utilization.
"AI X-ray technologies have the potential to redefine the global healthcare landscape, improving diagnosis, treatment outcomes, and accessibility. However, realizing this potential requires strategic investment in infrastructure, training, and regulatory alignment."
Potential Global Impact of AI X-ray Systems
Region | Opportunity | Challenge |
---|---|---|
Developed Countries | Increased diagnostic accuracy and reduced workload for healthcare providers. | High cost of implementation and integration with existing systems. |
Developing Countries | Improved access to advanced diagnostics in remote areas. | Lack of infrastructure and healthcare workforce training. |
Low-income Regions | Affordable AI systems could dramatically increase access to essential diagnostics. | Funding and support for widespread deployment remain a challenge. |