Deep Voice Learn
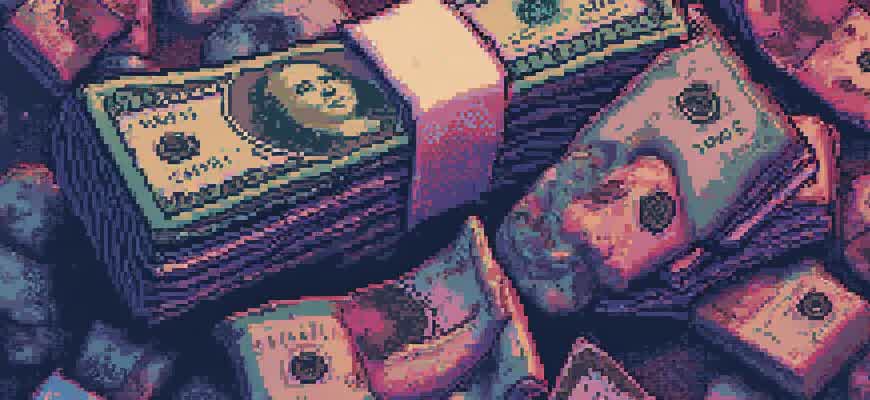
In recent years, advancements in artificial intelligence have significantly improved speech recognition technologies. One of the key developments in this field is the application of deep learning models, which have enhanced the quality and accuracy of voice synthesis and recognition systems.
Deep learning models can be broken down into several core components, each contributing to the overall functionality of voice recognition systems:
- Data Preprocessing: Cleaning and normalizing voice data to remove noise and enhance clarity.
- Feature Extraction: Identifying key characteristics of speech signals, such as pitch, tone, and rhythm.
- Model Training: Training deep neural networks on large datasets to detect patterns and improve accuracy over time.
"The ability to process vast amounts of audio data enables deep learning models to outperform traditional systems in terms of speech recognition and natural language processing."
Below is a table summarizing the components involved in the deep learning pipeline for voice recognition:
Component | Description |
---|---|
Data Collection | Gathering large, diverse datasets to represent various accents and languages. |
Model Design | Choosing the right neural network architecture to handle audio processing effectively. |
Training | Feeding data through the network to adjust weights and improve prediction accuracy. |
Testing & Validation | Evaluating the model's performance against real-world voice data. |