Speech Resynthesis
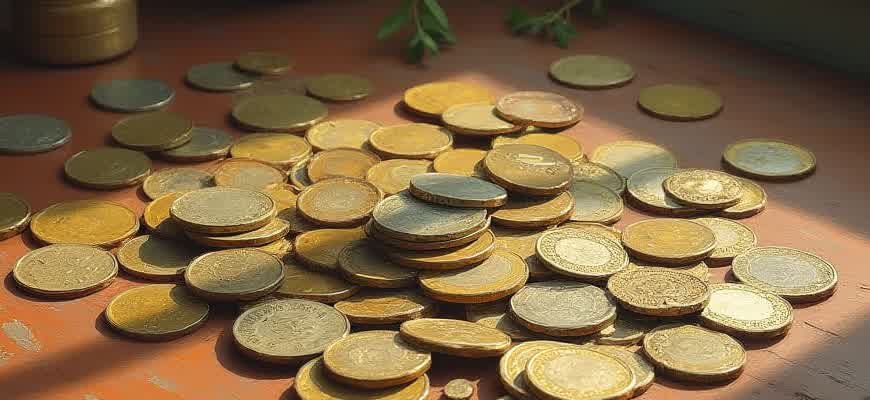
Speech resynthesis is the process of recreating a natural-sounding speech waveform from an input signal, often aiming to replicate or enhance certain aspects of human speech. This technology is vital in various fields, such as voice cloning, speech-to-speech translation, and assistive communication devices. The core challenge lies in accurately capturing the prosody, intonation, and emotional tone of a speaker while maintaining intelligibility.
Different methods are employed to achieve speech resynthesis, with some of the most notable being:
- Parametric methods: These rely on mathematical models to describe speech production.
- Concatenative methods: These use segments of prerecorded speech and stitch them together to form new utterances.
- Neural network-based methods: Leveraging deep learning models, this approach attempts to generate speech directly from a sequence of phonetic inputs.
Each approach has its advantages and trade-offs in terms of quality, computational demand, and flexibility.
Key Considerations in Speech Resynthesis:
- Preserving the naturalness of speech
- Ensuring high intelligibility
- Adapting to various speech styles and accents
To evaluate the performance of speech resynthesis methods, a variety of metrics are used. These may include:
Metric | Description |
---|---|
Naturalness | How closely the synthesized speech mimics natural human speech. |
Intelligibility | The clarity and understandability of the generated speech. |
Emotion Recognition | How accurately the resynthesized speech conveys emotional tones. |