Synthetic Users Ai
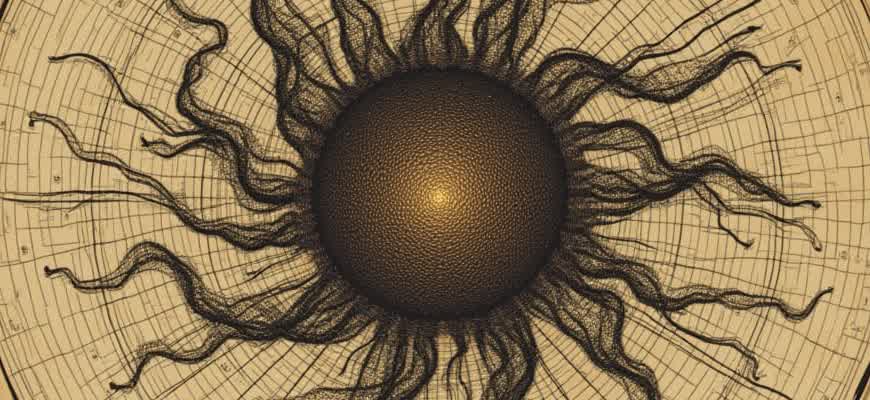
Synthetic users powered by artificial intelligence are virtual entities designed to mimic human behaviors and interactions within digital systems. These entities are programmed to simulate various user actions, such as browsing, shopping, or even engaging with social media platforms. The main purpose of these users is to replicate human-like activity, often for testing, development, or optimization purposes.
Here are some key applications of synthetic users in AI:
- Automated user testing and system performance evaluation
- Data generation for machine learning training sets
- Enhancing user experience by simulating interactions
While synthetic users can be beneficial in various contexts, they also present unique challenges:
- They can introduce bias if not carefully designed.
- There is potential for misuse in manipulating online environments.
- Ensuring their actions align with ethical standards is a growing concern.
Important: Synthetic users can significantly enhance the scalability of AI-driven platforms, but careful consideration must be given to their impact on data integrity and system reliability.
Promoting Synthetic Users AI: A Practical Approach
When integrating Synthetic Users AI into modern marketing strategies, it is essential to ensure that the approach aligns with the needs and preferences of both businesses and consumers. A successful promotion plan focuses on effectively demonstrating the capabilities of this technology, while also addressing potential concerns regarding data privacy and user experience.
To gain traction, the promotion strategy must be clear, transparent, and result-oriented. By leveraging real-life use cases and quantifiable outcomes, businesses can create a solid foundation for widespread adoption of Synthetic Users AI. This approach fosters trust and showcases the direct benefits for organizations and their customers.
Steps to Promote Synthetic Users AI
- Highlighting the benefits of AI-driven customer interactions in real-time.
- Providing clear demonstrations through case studies and client testimonials.
- Ensuring transparency around AI's role in data handling and decision-making processes.
- Utilizing educational content to break down complex AI concepts for the target audience.
Key Marketing Techniques
- Leverage Social Proof: Showcase successful integrations and endorsements from leading organizations.
- Content Strategy: Share insightful blog posts, videos, and webinars to explain the AI’s potential in enhancing user engagement.
- Targeted Advertising: Use data-driven ad campaigns that target industries likely to benefit from AI-driven user engagement.
Important Considerations
Consideration | Action |
---|---|
Data Privacy | Ensure clear consent protocols and data encryption methods are in place. |
AI Ethics | Develop and communicate ethical guidelines for the responsible use of AI technology. |
User Trust | Show transparency regarding AI’s decision-making processes to foster trust. |
Note: Promoting Synthetic Users AI successfully requires constant adaptation to technological developments and market demands. Staying ahead of trends will allow businesses to remain competitive in an ever-evolving digital landscape.
How Synthetic Users AI Enhances User Testing in Real-World Scenarios
Synthetic User AI has revolutionized the way companies approach user testing. By creating lifelike, artificial users, businesses can simulate complex interactions that reflect real-world behaviors, enabling them to test applications in diverse, unpredictable conditions. Unlike traditional user testing with human participants, synthetic users can operate at scale, mimicking various demographics and usage patterns, without the limitations of time or geographical constraints.
This technology enables faster iterations and more comprehensive testing, providing immediate feedback across multiple devices, environments, and user personas. With synthetic AI users, teams can test everything from the functionality of a website to the usability of complex mobile applications. This allows businesses to identify pain points early in the design process, ensuring a smoother user experience upon launch.
Key Benefits of Using Synthetic AI Users
- Scalability: Synthetic users can be generated in large numbers, enabling testing across different user groups and environments simultaneously.
- Cost-effectiveness: Unlike traditional user testing, there are no expenses associated with recruiting participants, making the process more budget-friendly.
- Real-time Feedback: Synthetic users interact with the system in real time, providing immediate insights into functionality and usability.
- Behavior Simulation: They can replicate user behavior across various demographics, creating realistic scenarios that would otherwise be difficult to simulate.
Practical Applications of Synthetic User AI
- Usability Testing: Synthetic users simulate real-world scenarios, helping to uncover usability flaws early in the design phase.
- Load Testing: By generating large volumes of synthetic users, developers can test how well a system handles high traffic and ensure scalability.
- Cross-Platform Compatibility: These users can interact with applications across different devices, ensuring consistent experiences across platforms.
Comparing Traditional User Testing vs. Synthetic User AI
Aspect | Traditional Testing | Synthetic User AI |
---|---|---|
Cost | High (due to recruitment and compensation) | Low (no recruitment required) |
Speed | Slow (due to scheduling and recruitment) | Fast (testing can begin immediately) |
Realism | Varies (depends on the participant's engagement) | High (AI replicates diverse, real-life behaviors) |
“Synthetic User AI provides the ability to test user experience on a massive scale, delivering valuable insights with minimal time and resources.”
Enhancing Customer Segmentation Using Synthetic Users for Precise Targeting
With the growing complexity of consumer behavior, businesses face significant challenges in accurately segmenting their audiences. Traditional methods often fall short due to limited data or biases, making it harder to create effective marketing strategies. Leveraging synthetic users, or AI-generated personas, can bridge this gap by providing a richer and more diverse dataset for analysis.
By simulating various user behaviors and preferences, synthetic users allow companies to experiment with new targeting strategies, optimize marketing campaigns, and discover hidden patterns in customer interactions. This approach goes beyond simple demographic segmentation and enables a deeper understanding of behavioral and psychographic trends.
Key Benefits of Using Synthetic Users for Customer Segmentation
- Improved Precision: By generating realistic customer profiles, businesses can target niche segments more effectively, resulting in higher conversion rates.
- Cost Efficiency: Instead of relying on costly market research or focus groups, synthetic users provide a cost-effective alternative to test different strategies.
- Scalability: Synthetic user models can quickly scale to simulate large populations, allowing businesses to assess a wider range of scenarios.
"Using synthetic users allows us to test a variety of market segments in a controlled environment, providing valuable insights before launching a full-scale campaign."
Steps for Implementing Synthetic Users in Customer Segmentation
- Data Collection: Gather data from existing customer profiles, interactions, and behaviors.
- Persona Creation: Use AI algorithms to generate diverse synthetic user profiles based on this data.
- Behavioral Simulation: Test how these synthetic users interact with different marketing channels and content.
- Segmentation and Analysis: Analyze the results to identify patterns, trends, and preferences for more effective targeting.
Example: Customer Segmentation with Synthetic Users
Segment | Key Characteristics | Targeting Strategy |
---|---|---|
High-Spending Shoppers | Frequent purchases, high-income, luxury products | Personalized offers, premium content |
Price-Sensitive Buyers | Occasional purchases, budget-conscious, discount seekers | Special promotions, limited-time discounts |
Brand Loyalists | Frequent brand interactions, repeat purchases | Exclusive rewards, loyalty programs |
Optimizing Stress Testing with AI-Driven Synthetic Users
In today's fast-paced digital landscape, ensuring the reliability and scalability of your infrastructure is critical. Traditional stress testing methods can be limited by the ability to replicate real-world user behavior at scale. By employing AI-generated synthetic users, organizations can create highly dynamic, varied loads on their systems, mimicking actual user interactions with exceptional accuracy. This enables businesses to pinpoint vulnerabilities that would otherwise go unnoticed under typical test scenarios.
Leveraging AI-powered synthetic users for load and performance testing provides a deeper understanding of system behavior under stress. These virtual users can simulate complex user journeys, interactions, and traffic patterns that mimic a wide range of real-world conditions, allowing teams to assess how their digital infrastructure handles extreme stress. Below are some of the ways in which AI-enhanced synthetic users improve testing outcomes:
Advantages of AI-Generated Synthetic Users
- Realistic Traffic Simulation: Synthetic users can replicate diverse behaviors, including browsing patterns, clicks, and transactions, ensuring a comprehensive test environment.
- Scalability: AI can generate thousands or millions of synthetic users, creating load scenarios far beyond the capacity of manual testing efforts.
- Adaptive Stress Testing: AI can adjust user patterns dynamically based on system responses, enabling more precise and evolving tests.
- Increased Accuracy: These users mimic not just quantity, but also the complexity of real-world interactions, ensuring tests are more reflective of actual usage.
Key Benefits for Digital Infrastructure Testing
- Early Detection of Weaknesses: Stress testing with synthetic users identifies potential bottlenecks or vulnerabilities in the system that could compromise performance under real-world conditions.
- Enhanced Resource Allocation: AI-generated loads help teams understand resource consumption during peak traffic periods, allowing for more informed capacity planning.
- Better User Experience Insights: Through simulated high-traffic scenarios, businesses can observe how performance degradation impacts end-user experience, leading to improved optimization efforts.
"By using AI-based synthetic users, companies can push their infrastructure to the limit, ensuring that it will perform effectively and efficiently under the most demanding conditions."
Example: Testing Digital Infrastructure with Synthetic Users
Test Type | Outcome |
---|---|
Scalable Load Simulation | Ability to simulate thousands of concurrent users without manual input. |
Behavioral Interaction Analysis | Identifies potential user journey friction points, leading to UX improvements. |
Peak Traffic Load | Reveals system performance and resilience during high-stress periods. |
Cost Reduction Strategies: Leveraging Synthetic Users for Scalable Data Generation
In the field of data-driven decision-making, the ability to generate large volumes of high-quality data is crucial for testing algorithms, improving machine learning models, and conducting user experience research. Traditionally, obtaining vast amounts of real user data involves substantial costs in recruitment, incentives, and operational logistics. However, the emergence of synthetic users has revolutionized this process, offering a cost-effective and scalable alternative.
Synthetic users, powered by artificial intelligence, simulate real user behaviors, interactions, and preferences. They can be created on demand, providing a highly customizable and reusable data source. These users are particularly valuable in large-scale data generation tasks, enabling businesses to run extensive tests, model complex scenarios, and collect diverse data sets without the need for extensive physical user involvement.
Key Benefits of Using Synthetic Users for Cost Reduction
- Scalability: Synthetic users can be generated in virtually unlimited numbers, providing datasets that are impossible or impractical to collect using real users.
- Lower Operational Costs: Since there is no need for physical recruitment or payment to participants, businesses can significantly reduce data acquisition expenses.
- Faster Data Generation: Unlike real-world data collection, which can take weeks or months, synthetic users can provide vast amounts of data in a fraction of the time.
Cost-Effective Use Cases for Synthetic Users
- User Testing: Companies can create synthetic users to simulate a wide range of behaviors, enabling rapid testing of website features, app interfaces, or e-commerce flows.
- Model Training: Generating diverse data with synthetic users helps machine learning models train more efficiently, improving performance in real-world applications.
- Load Testing: For performance optimization, synthetic users can simulate high traffic loads, helping to identify potential bottlenecks or system failures before they affect real users.
"Synthetic users enable cost-effective, scalable data generation for tasks that require large, diverse datasets without the logistical and financial burdens of traditional methods."
Example of Cost Reduction in Data Generation
Traditional Approach | Synthetic Users Approach |
---|---|
Recruitment, incentives, and operational costs for real users. | No recruitment or participant incentives needed, saving on logistics and operational overhead. |
Time-intensive data collection processes. | Instant data generation with customizable parameters, reducing project timelines. |
Limited scalability with real user involvement. | Unlimited scalability for testing and data generation, tailored to specific needs. |
How to Seamlessly Add Synthetic User AI into Your Marketing Automation Systems
Integrating synthetic users powered by artificial intelligence into your marketing automation processes can significantly optimize your workflow. These virtual agents simulate real user behavior, enabling businesses to analyze interactions and test various strategies without relying on actual customers. By combining synthetic users with automation tools, brands can enhance their marketing campaigns with more accurate data and better segmentation.
The integration process involves configuring the AI to mimic user journeys, personalize content delivery, and generate insights that can inform future decisions. This allows businesses to perform A/B testing, optimize email sequences, and forecast the impact of different marketing strategies without the need for a large pool of real users. Below are the essential steps and considerations when incorporating synthetic users into your existing tools.
Steps to Implement Synthetic Users AI in Your Marketing Workflow
- Identify Automation Tools Compatible with AI: Start by evaluating your current marketing platforms, such as CRM systems, email automation tools, and analytics platforms, to ensure they support AI integration.
- Define User Behavior Models: Determine the key behaviors you want the synthetic users to replicate (e.g., browsing patterns, purchase decisions, engagement with content).
- Train the AI: Use historical data to train the synthetic users, ensuring they simulate realistic customer interactions. This can include analyzing past purchase histories, click-through rates, and customer feedback.
- Monitor and Adjust: Once the synthetic users are integrated, monitor their interactions with the system. Fine-tune the models based on performance to improve accuracy and relevancy.
Integrating synthetic users allows you to enhance customer segmentation, test multiple strategies, and improve your marketing ROI by leveraging AI-driven simulations.
Common Tools for Integrating Synthetic User AI
Marketing Tool | Integration Feature | AI Compatibility |
---|---|---|
Email Marketing Platforms | Automated Campaigns, A/B Testing | High |
CRM Systems | Customer Data Analysis, Lead Scoring | Moderate |
Analytics Platforms | User Behavior Insights, Reporting | High |
Personalization at Scale: Tailoring Synthetic User Actions for Precision Marketing
In the world of digital marketing, delivering personalized experiences is crucial for maximizing engagement and conversion. With the rise of synthetic users powered by AI, it has become easier to scale personalized campaigns and create interactions that feel deeply relevant to target audiences. These virtual entities mimic real user behavior and can be customized to represent specific segments, allowing brands to test different scenarios and optimize their messaging at scale. The flexibility and adaptability of synthetic users provide unparalleled opportunities for marketers to fine-tune their strategies and increase campaign effectiveness.
Leveraging AI-generated users for large-scale personalization enables companies to address highly specific needs and interests, making each interaction feel unique. By analyzing vast datasets and using machine learning techniques, synthetic users can be tailored to reflect specific behaviors, preferences, and goals. This level of customization is not only cost-effective but also highly efficient, ensuring that marketing efforts resonate with the right audiences at the right time.
Key Elements of Tailoring Synthetic User Behavior
- Demographic Targeting: Synthetic users can be programmed to reflect various demographic groups such as age, location, gender, and occupation. This allows for precise targeting of campaigns based on real-world data.
- Behavioral Patterns: By analyzing user actions, synthetic users can replicate specific behaviors, such as browsing history, click-through rates, or product preferences, ensuring that marketing efforts align with consumer habits.
- Interaction Simulations: These virtual entities can engage with content in ways that simulate authentic user actions, providing valuable insights into how real users might respond to different strategies.
Benefits of Scaling Personalized Campaigns with Synthetic Users
- Enhanced Testing: Marketers can test different content variations, landing pages, and call-to-action strategies with synthetic users, ensuring they deliver the most effective campaign elements before launching at scale.
- Cost Efficiency: Rather than relying on real-world user groups for testing, synthetic users allow for large-scale experimentation without the associated costs of conducting traditional focus groups or A/B testing.
- Data-Driven Insights: By observing how synthetic users interact with campaigns, marketers can gather valuable data that informs future strategy and refines targeting models.
"Using synthetic users not only accelerates the process of personalization but also helps in refining digital marketing strategies with a level of precision that traditional methods cannot match."
Practical Applications in Campaigns
Application Area | Impact |
---|---|
Product Recommendations | Synthetic users allow for testing personalized product suggestions based on browsing and purchase behavior. |
Email Campaigns | Tailoring email content based on synthetic user profiles can increase open rates and engagement. |
Social Media Ads | Targeting ads to specific synthetic user segments ensures more relevant and compelling ads. |
Ensuring Data Privacy and Security When Using Synthetic Users in Your Projects
As synthetic users are increasingly utilized in various projects to simulate real-world interactions and test systems, ensuring the privacy and security of the data involved becomes crucial. These artificial profiles often rely on large datasets, which can inadvertently expose sensitive information or create vulnerabilities if not handled correctly. Safeguarding this data requires a multifaceted approach that combines encryption, data anonymization, and proper access control measures.
When dealing with synthetic users, it’s important to implement best practices for data protection throughout the lifecycle of these profiles. Not only does this help mitigate potential security risks, but it also ensures that the synthetic data used does not violate any privacy regulations or lead to the unintended exposure of personal information.
Key Measures for Ensuring Privacy and Security
- Data Anonymization: Before synthetic users are created, ensure that any real-world data used to generate profiles is anonymized. This eliminates the possibility of tracing the synthetic users back to real individuals.
- Secure Data Storage: All synthetic user data should be stored in encrypted databases to prevent unauthorized access. Employ state-of-the-art encryption techniques to protect sensitive information.
- Access Control: Implement strict access controls to limit who can view or modify synthetic user data. Use multi-factor authentication (MFA) and role-based access to ensure only authorized personnel can interact with the data.
Best Practices for Maintaining Data Integrity
- Regular Audits: Conduct regular security audits to identify and address potential vulnerabilities in your data handling processes.
- Data Minimization: Only collect and store the minimum amount of data required for the intended purpose of synthetic users. This reduces the risk of exposing unnecessary information.
- Transparency: Clearly document the methods and policies used to protect data privacy and security, ensuring that all stakeholders understand the measures in place.
"By anonymizing data and employing robust encryption techniques, organizations can greatly reduce the risk of exposing sensitive information while still leveraging synthetic users for testing and research purposes."
Comparison of Data Protection Techniques
Technique | Benefits | Challenges |
---|---|---|
Anonymization | Reduces risk of identifying real individuals, supports compliance with privacy regulations | May reduce the accuracy of synthetic users for certain types of testing |
Encryption | Ensures data is protected from unauthorized access | Can introduce performance overheads, requires proper key management |
Access Control | Limits exposure to sensitive data, supports compliance with security protocols | Requires ongoing management and can lead to operational bottlenecks if not implemented correctly |