Ai Voice Accent Recognition
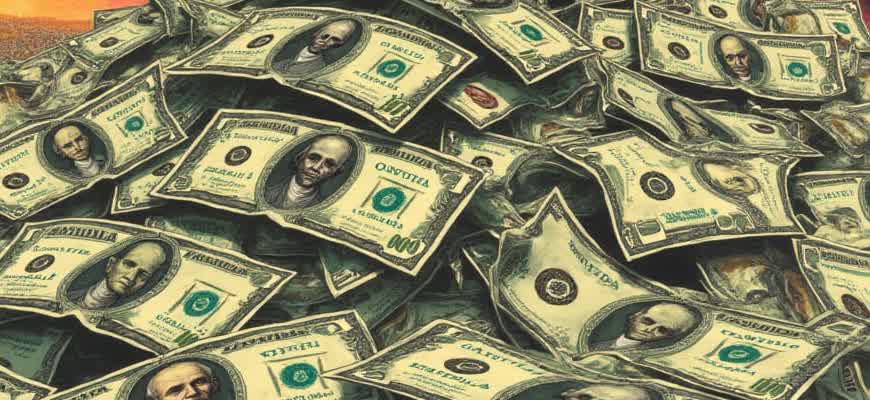
Recent advancements in AI-driven voice analysis have introduced innovative methods to identify and classify speech accents. By leveraging machine learning algorithms, these systems can detect subtle variations in pronunciation, rhythm, and intonation, which are often unique to specific regions or social groups.
The core components that contribute to accent detection include phonetic features, voice pitch, and speech rate. By training neural networks on large datasets, AI models can differentiate between various accent types with impressive accuracy. The process involves analyzing patterns in audio samples and comparing them to a predefined database of known accents.
Key Insight: AI accent recognition models not only recognize regional accents but also capture sociolects, helping in a more comprehensive analysis of speech.
Some of the major steps in AI accent recognition are outlined below:
- Data Collection: Gathering a diverse range of speech samples representing various accents.
- Feature Extraction: Identifying unique acoustic features in the speech such as tone, pitch, and speech duration.
- Model Training: Using machine learning techniques to train the model on the collected data.
- Recognition and Classification: The trained model categorizes the accent from the input speech.
In addition to the primary acoustic features, several external factors can influence the accuracy of accent detection, such as:
Factor | Impact on Recognition |
---|---|
Background Noise | Reduces model accuracy due to interference with speech signals. |
Speech Clarity | Higher clarity leads to better recognition, while unclear speech can hinder detection. |
Language Proficiency | Fluency in the language can affect accent detection, especially for non-native speakers. |
AI Voice Accent Recognition: A Practical Approach
Accent recognition in voice AI has gained significant attention due to the increasing need for accurate speech processing across diverse linguistic backgrounds. By analyzing variations in pronunciation, rhythm, and intonation, AI systems can identify regional or cultural accents. The challenge lies in developing models that can efficiently handle these variations without compromising on the quality of transcription or speech recognition.
Implementing accent recognition requires a combination of machine learning techniques, acoustic modeling, and large-scale data. This practical approach involves understanding the phonetic patterns specific to various accents, as well as continuously updating models to adapt to evolving speech characteristics. Key strategies include collecting diverse datasets, applying deep learning algorithms, and integrating natural language processing (NLP) for better contextual understanding.
Approach to Accent Recognition
- Data Collection: Gathering diverse, annotated speech samples from a variety of accent groups.
- Feature Extraction: Identifying distinctive acoustic features such as pitch, tone, and speech rate.
- Model Training: Using supervised learning to train algorithms to differentiate between accents based on acoustic features.
- Model Testing: Evaluating the system on previously unseen accents to ensure generalization and accuracy.
Key Techniques Used
- Deep Neural Networks (DNN): Leverage large neural networks to model complex accent variations.
- Recurrent Neural Networks (RNN): Capture temporal dependencies in speech for better accent differentiation.
- Transfer Learning: Adapt pre-trained models to new accent recognition tasks with minimal data.
“The success of accent recognition is closely tied to the quality and diversity of the training data. More diverse datasets lead to better generalization, ensuring accuracy in real-world applications.”
Challenges and Future Directions
Challenge | Solution |
---|---|
Speech Variability | Continuous updates and augmentation of training datasets. |
Accent Confusion | Advanced modeling techniques like multi-task learning to distinguish similar accents. |
Real-time Processing | Optimization of AI algorithms for faster, real-time accent recognition. |
Understanding the Technology Behind AI Voice Accent Recognition
AI-driven voice accent recognition relies on sophisticated algorithms that analyze speech patterns to identify regional or cultural variations in pronunciation. This technology is built upon natural language processing (NLP) and machine learning models, which enable the system to distinguish between different accents and dialects. These systems are trained using vast amounts of speech data, allowing them to capture nuanced differences in pronunciation, intonation, and rhythm.
At the core of this technology lies acoustic analysis. By breaking down sound waves into their frequency and amplitude components, AI systems can detect minute differences between how various sounds are articulated. This analysis is then used to create a model that can match new input with known accent patterns, making accent recognition possible even in real-time conversations.
Key Components of AI Voice Accent Recognition
- Data Collection: Large datasets of speech from diverse geographical regions are collected to represent a wide variety of accents.
- Acoustic Features Extraction: Speech signals are processed to extract features such as pitch, tone, and rhythm that are characteristic of specific accents.
- Machine Learning Algorithms: Models such as deep learning networks or decision trees are trained to classify accents based on extracted features.
Important: The effectiveness of accent recognition models depends on the diversity and quality of the training data, as biases or gaps in the dataset can result in less accurate identification.
How AI Recognizes Accents
- Preprocessing: Audio input is first cleaned and normalized to reduce noise and improve clarity.
- Feature Extraction: The AI extracts phonetic features that are unique to the accent.
- Classification: The features are then used to match the speech with a known accent model.
- Post-Processing: The final output is refined to improve accuracy and reliability.
Accent Type | Key Characteristics |
---|---|
British English | Distinct vowel sounds, non-rhotic pronunciation |
American English | Stronger rhotic sounds, flat intonation |
Australian English | Combination of British and American, rising intonation at the end of sentences |
How AI-Based Accent Detection Enhances Customer Support Solutions
In today's globalized world, customer support teams deal with clients from various regions, each with unique accents. Traditional systems often face challenges in accurately interpreting these accents, leading to inefficiencies and miscommunication. AI-powered voice accent recognition technology provides a solution by enabling systems to identify and process various accents with high precision, improving the overall customer experience. This capability ensures that support agents can focus on solving problems rather than clarifying misunderstood speech.
By leveraging machine learning algorithms, AI voice recognition tools adapt to diverse speech patterns and regional nuances. These tools analyze linguistic features like pronunciation, intonation, and rhythm, allowing support systems to automatically adjust to the speaker's accent. As a result, communication becomes smoother, and customers experience faster and more accurate responses from support agents.
Key Benefits of AI Voice Accent Recognition in Customer Support
- Enhanced Understanding: AI models learn to recognize diverse accents, reducing the chance of misunderstanding and misinterpretation during customer interactions.
- Faster Response Times: With real-time recognition, support teams can immediately identify the issue, ensuring quicker resolutions.
- Improved Customer Satisfaction: Accurate recognition leads to more effective communication, leaving customers feeling heard and valued.
- Scalable Solution: AI systems can handle increasing volumes of diverse customer inquiries without compromising on performance.
How It Works
- Data Collection: Speech samples are collected from diverse accents to build a comprehensive dataset.
- Model Training: Machine learning algorithms are trained on this data, enabling them to identify and interpret different accent features.
- Real-Time Application: During interactions, the system continuously adapts, detecting and processing the accent in real-time for accurate interpretation.
- Continuous Improvement: As the system interacts with more customers, it refines its ability to understand new accents and dialects.
"AI-powered voice recognition not only streamlines customer support interactions but also fosters a more inclusive environment, ensuring that no accent goes unnoticed or misunderstood."
Impact on Operational Efficiency
Metric | Before AI Implementation | After AI Implementation |
---|---|---|
First Call Resolution Rate | 65% | 85% |
Customer Wait Time | 7 minutes | 3 minutes |
Accuracy of Speech Recognition | 75% | 95% |
Integrating AI-Based Accent Recognition in Global Business Operations
In the context of multinational organizations, communication is key to effective collaboration. However, linguistic diversity can lead to challenges in understanding, especially when employees or clients come from various regions with distinct accents. AI-driven accent recognition technologies offer a practical solution by enabling smoother interactions across different languages and dialects, thus improving overall operational efficiency. By analyzing speech patterns, tone, and intonation, these systems can identify regional accents, allowing for tailored language processing.
Implementing AI voice accent recognition into business environments fosters a more inclusive and efficient workplace. This technology enhances customer support, improves cross-cultural communication, and allows for better data analytics on voice-based interactions. The systems are capable of accurately recognizing a wide range of accents, adapting to the nuances of different dialects, and overcoming potential barriers caused by accent-related misunderstandings.
Benefits for Multinational Business Environments
- Improved Communication: AI accent recognition helps to ensure that speech is accurately transcribed and understood, regardless of accent.
- Enhanced Customer Experience: By adapting to regional speech patterns, AI can provide more personalized customer support, increasing satisfaction.
- Efficiency Gains: Real-time translation and transcription of regional accents streamline operations and reduce miscommunications.
- Market Expansion: Recognizing diverse accents allows companies to enter new markets and reach a broader, more global audience.
Challenges and Considerations
- Data Privacy and Security: Ensuring that voice data used for accent recognition is securely stored and processed is a priority to avoid breaches.
- Accuracy Across Diverse Accents: AI systems must be continuously trained to handle various regional dialects and evolving language trends.
- Integration with Existing Systems: Businesses need to integrate accent recognition systems smoothly with existing communication platforms for optimal performance.
Key Insights
"AI voice accent recognition is transforming the way businesses operate across borders, ensuring seamless communication and greater inclusivity in every aspect of the organization."
Table: Comparison of Accent Recognition Technologies
Technology | Accuracy | Implementation Complexity | Cost |
---|---|---|---|
AI-based Speech Recognition | High | Moderate | Medium |
Traditional NLP Systems | Moderate | Low | Low |
Deep Learning Algorithms | Very High | High | High |
Enhancing Language Learning with AI-Powered Accent Analysis
Accent recognition through artificial intelligence is transforming the way we approach language acquisition. Traditional language learning often emphasizes grammar and vocabulary, but it falls short in addressing the nuances of pronunciation, which can significantly affect communication. AI-based accent analysis tools provide a more holistic approach to mastering a language, offering learners the ability to fine-tune their pronunciation skills in a personalized manner.
By utilizing advanced speech recognition algorithms, these AI systems can identify subtle differences in pronunciation, even detecting regional accents. This technology enables learners to receive immediate, actionable feedback, helping them align their speech with native speakers. This form of feedback enhances the learner’s ability to assimilate the rhythm, intonation, and phonetic nuances of the target language more effectively than traditional methods.
Key Benefits of AI in Accent Analysis for Language Learning
- Real-time feedback: Learners can receive instant corrections, improving the speed of language acquisition.
- Personalized learning: AI systems tailor feedback based on the individual’s specific accent errors and challenges.
- Wide accessibility: AI-powered tools make language learning more accessible to people from diverse linguistic backgrounds.
How AI Improves Pronunciation Mastery
- Accent Identification: AI tools analyze the user's pronunciation and compare it to a database of native speech patterns to identify any mismatches.
- Contextual Feedback: Rather than just pointing out errors, AI offers context for why certain sounds or rhythms are incorrect, aiding deeper understanding.
- Continuous Improvement: With ongoing practice, AI can track progress and adapt feedback, ensuring the learner continuously improves.
Comparison: Traditional vs. AI-Enhanced Learning
Aspect | Traditional Learning | AI-Powered Learning |
---|---|---|
Feedback Speed | Delayed, often reliant on instructor's availability | Instant, automated feedback available anytime |
Personalization | Generalized advice, may not address specific issues | Tailored to the individual’s unique accent and progress |
Engagement | Dependent on instructor interaction and class environment | Interactive, gamified elements for sustained interest |
AI tools make learning a new language more interactive, personalized, and efficient, focusing not just on words but on how they are spoken.
Optimizing Speech Recognition Accuracy through Accent Adaptation
Modern speech recognition systems face significant challenges in accurately transcribing spoken language due to the diverse range of accents within a language. Effective adaptation to these accents is critical in improving the overall system performance. Accent variation not only influences pronunciation but also affects speech rhythm, intonation, and speech rate, all of which can lead to higher recognition errors when left unaddressed. Integrating accent adaptation into speech recognition technology can substantially improve its accuracy across different regional speakers.
In order to optimize the accuracy of speech recognition systems, developers must incorporate features specifically designed to handle different accents. These features should focus on adjusting the system's phonetic model to accommodate regional speech patterns, as well as enhance the ability to process subtle differences in pronunciation. Below are some key strategies for improving speech recognition accuracy through accent adaptation:
Key Strategies for Accent Adaptation
- Phonetic Model Tuning: Adjusting the phonetic models to include common accent-based phonemes can help reduce transcription errors.
- Data Augmentation: Expanding training datasets with varied accents and speech patterns ensures better representation of diverse speech.
- Transfer Learning: Using pre-trained models on large datasets and fine-tuning them with accent-specific data enhances system performance.
Accent adaptation in speech recognition is not a one-size-fits-all approach; it requires continuous refinement and integration of diverse speaker data to ensure high accuracy across all accent groups.
Techniques to Enhance Recognition for Accented Speech
- Speech Signal Processing: Improve the clarity of speech signals by employing noise reduction and echo cancellation techniques to minimize errors caused by acoustic variations in different accents.
- Dynamic Acoustic Modeling: Use real-time adjustments to the acoustic model to dynamically adapt to different accents during speech input.
- Accent-Specific Language Models: Developing language models trained specifically on the dialects or regional speech patterns improves the recognition system's ability to predict contextual speech correctly.
Technique | Description |
---|---|
Phonetic Model Tuning | Adapts the model to include accent-specific phonemes and sounds. |
Data Augmentation | Expands training data to represent a variety of accents and speaking styles. |
Transfer Learning | Uses pre-trained models and adapts them to regional speech variations. |
AI-based Accent Recognition in Transcription Services: Advantages and Challenges
In recent years, AI technology has made significant strides in improving transcription services, particularly through the integration of voice recognition systems that can accurately identify and transcribe various accents. This innovation plays a crucial role in enhancing accessibility and efficiency for individuals and businesses, enabling them to transcribe audio content more quickly and accurately. With the ability to recognize different accents, transcription services are now able to cater to a global audience, improving their overall performance and user satisfaction.
Despite its advancements, AI voice recognition for accents comes with its own set of challenges. While the technology has made substantial improvements, issues such as misinterpretation of speech patterns, regional dialects, and background noise still pose significant barriers. These obstacles can lead to errors in transcription and create inconsistencies, making it important to understand both the benefits and limitations of AI-driven accent recognition systems.
Benefits of AI-based Accent Recognition in Transcription
- Improved Accessibility: AI systems can recognize a wide variety of accents, making transcription services more inclusive for people from different linguistic backgrounds.
- Increased Efficiency: The ability to quickly and accurately transcribe voice recordings in multiple accents reduces the time spent on manual corrections and increases overall productivity.
- Better Customer Satisfaction: Users from different regions benefit from improved transcription accuracy, resulting in fewer mistakes and a more reliable service.
Challenges of AI-based Accent Recognition in Transcription
- Accent Variability: Despite advancements, AI models may still struggle with recognizing certain regional accents or dialects, leading to potential inaccuracies in transcriptions.
- Background Noise: External noise in the audio can distort the clarity of the accent, making it difficult for AI systems to deliver accurate transcriptions.
- Continuous Learning Requirement: Accent recognition models need constant updates and training to stay effective across a broad range of languages and accents.
"AI-driven accent recognition in transcription services significantly boosts global communication and accessibility, but it requires ongoing refinement to handle the complexities of diverse speech patterns."
Comparison of AI Accuracy by Accent Type
Accent Type | Recognition Accuracy |
---|---|
Standard American English | 95% |
British English | 90% |
Australian English | 85% |
Indian English | 80% |
Reducing Miscommunication in Global Teams Using Accent Recognition Tools
In a globalized business environment, teams often consist of individuals from various linguistic backgrounds. Effective communication is essential, but accents can sometimes lead to misunderstandings or confusion. Accent recognition technology offers a promising solution to bridge communication gaps in diverse teams by analyzing speech patterns and identifying potential areas where clarity may be needed.
Accent recognition tools can significantly enhance communication by providing real-time feedback and suggestions, helping to ensure that everyone in a team is on the same page. This is particularly crucial in remote work settings, where face-to-face interactions are limited, and the risk of misinterpretation increases. By implementing these tools, organizations can foster a more inclusive and efficient work environment, where language barriers are minimized.
Key Benefits of Accent Recognition in Teams
- Improved Understanding: By detecting speech variations, the tool can help individuals adjust their pronunciation or clarify words, reducing confusion.
- Enhanced Inclusivity: Teams from diverse regions can communicate more comfortably without fear of being misunderstood or judged for their accent.
- Increased Productivity: With fewer communication barriers, team members can collaborate more effectively, leading to faster decision-making and problem-solving.
How Accent Recognition Tools Work
- Speech Analysis: The tool captures speech and analyzes phonetic features unique to different accents.
- Real-Time Feedback: If a potential misunderstanding is detected, the system can prompt suggestions for clearer speech or alternative phrasing.
- Adaptation Over Time: As the tool learns from continuous interactions, it can improve its accuracy, providing more personalized assistance.
"By integrating accent recognition, businesses create a more harmonious and productive work environment, ensuring that accent differences don't become barriers to collaboration."
Example of Accent Recognition Impact on Global Teams
Country | Common Accent Challenges | Accent Recognition Solutions |
---|---|---|
United States | Varied regional dialects (e.g., Southern, New York) | Real-time suggestions for clarification of regional terms or pronunciations |
India | Non-native English accents | Enhanced recognition of Indian English patterns to reduce miscommunication |
United Kingdom | Multiple accents across regions (e.g., Cockney, RP) | Speech modification tips to help team members understand different regional accents |