Ai Synthetic Biology
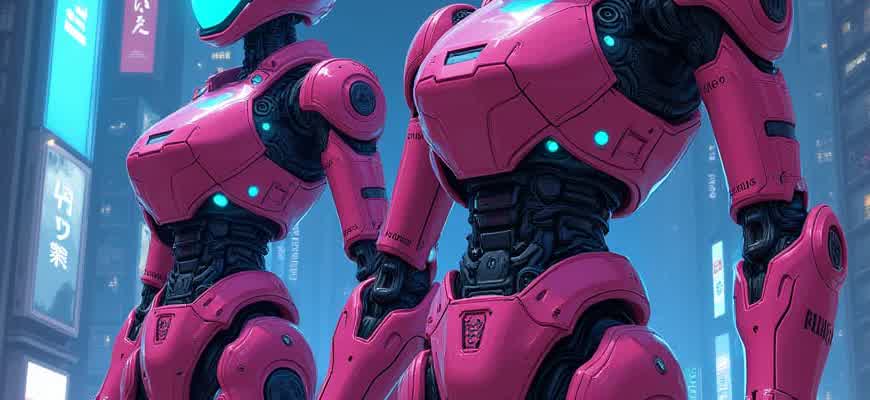
Artificial intelligence is rapidly transforming synthetic biology, enhancing the speed and precision with which biological systems are designed and engineered. AI algorithms enable researchers to analyze vast datasets, identify patterns, and optimize biological processes that would otherwise be computationally infeasible. With AI, scientists are now able to develop custom organisms and biological systems for various applications, from drug production to environmental remediation.
Key Areas of AI Integration in Synthetic Biology:
- Genetic Design Optimization: AI is used to predict gene sequences that will produce desired traits in organisms.
- Metabolic Pathway Engineering: AI models are used to simulate and optimize metabolic pathways for industrial-scale production.
- Biological Data Analysis: Machine learning techniques help extract actionable insights from large biological datasets.
AI Applications and Impact:
AI's ability to model complex biological systems allows for faster, more accurate predictions and solutions to biological challenges, reducing the need for trial and error in laboratory settings.
AI Tools and Techniques Used in Synthetic Biology:
Tool/Technique | Application |
---|---|
Deep Learning | Pattern recognition in genomic sequences, optimization of protein structures. |
Reinforcement Learning | Optimizing metabolic pathways in engineered microorganisms. |
Natural Language Processing | Processing and interpreting scientific literature for discovery of new biological insights. |
AI-Driven Innovation in Synthetic Biology: Revolutionizing Biotechnology
Integrating artificial intelligence (AI) into synthetic biology is rapidly reshaping the landscape of biotechnology. By leveraging AI's powerful data-processing capabilities, researchers are now able to design and engineer biological systems with unprecedented precision. The combination of AI and synthetic biology enables the development of new biological circuits, microorganisms, and therapies, pushing the boundaries of what is possible in fields like medicine, agriculture, and environmental sustainability.
The core strength of AI in synthetic biology lies in its ability to analyze vast amounts of biological data, identify patterns, and predict outcomes. This synergy not only accelerates the design of synthetic organisms but also enhances their functionality, optimizing their ability to perform specific tasks. As AI tools become more sophisticated, the efficiency and accuracy of synthetic biology processes improve, leading to faster innovations and applications across industries.
Key Applications of AI in Synthetic Biology
- Gene Editing and Design: AI algorithms optimize CRISPR techniques, enabling precise genetic modifications and minimizing off-target effects.
- Metabolic Engineering: AI is used to design microorganisms capable of producing biofuels, pharmaceuticals, and other valuable chemicals.
- Protein Folding and Drug Discovery: Machine learning models predict protein structures and interactions, aiding in the discovery of novel drugs and vaccines.
Impact of AI Integration on Biotechnology
- Enhanced Precision: AI models increase the accuracy of genetic designs, reducing the trial-and-error process in synthetic biology.
- Accelerated Discovery: AI enables faster identification of useful biological pathways and systems, cutting down research time and costs.
- Personalized Medicine: AI-driven algorithms facilitate the development of tailor-made therapeutic solutions based on individual genetic profiles.
"AI's integration into synthetic biology opens new frontiers in biotechnology, driving more sustainable solutions and creating transformative healthcare possibilities."
Challenges and Future Directions
Challenge | Potential Solutions |
---|---|
Data Complexity | Advanced machine learning models capable of handling and interpreting complex biological datasets. |
Ethical Considerations | Developing guidelines and regulations to ensure responsible AI use in genetic manipulation. |
Integration with Existing Systems | Building interoperable tools that bridge AI platforms with traditional biotechnology research methods. |
How Artificial Intelligence is Transforming Synthetic Biology: Key Applications and Advantages
Synthetic biology, a rapidly evolving interdisciplinary field, benefits immensely from the integration of artificial intelligence (AI). AI enables more precise and efficient manipulation of biological systems, accelerating the design, development, and optimization of synthetic organisms. By leveraging machine learning algorithms, AI can predict the behavior of engineered cells and organisms, vastly improving the speed and accuracy of experiments in this domain. This synergy opens new frontiers for innovation, particularly in areas such as drug development, biofuels, and environmental remediation.
The intersection of AI and synthetic biology introduces several game-changing opportunities, from automating complex biological processes to providing insights that would otherwise be difficult to obtain. One of the most notable applications is the ability to predict genetic modifications and their outcomes. AI’s capacity for processing large datasets allows for a more comprehensive understanding of genetic networks and metabolic pathways, laying the groundwork for more efficient and sustainable biotechnological solutions.
Applications of AI in Synthetic Biology
- Genetic Design and Optimization: AI assists in designing genes and synthetic circuits with a high degree of precision. Machine learning models analyze vast genetic data, enabling the creation of optimized organisms tailored for specific functions, such as drug production or agricultural improvement.
- Metabolic Pathway Prediction: AI can model and predict the behavior of metabolic pathways, helping researchers to design organisms that can more efficiently produce biofuels or pharmaceuticals by altering their metabolic networks.
- Automation of Laboratory Processes: AI-powered robotics and automated systems streamline experimental workflows, reducing human error and allowing for more rapid testing and iteration in synthetic biology labs.
- Protein Folding and Design: AI can predict the structure of proteins based on genetic sequences, enhancing the design of enzymes or therapeutic proteins for industrial and medical applications.
Benefits of Integrating AI with Synthetic Biology
- Increased Efficiency: AI significantly speeds up the process of designing and testing genetic modifications by automating routine tasks and reducing time-consuming trial-and-error approaches.
- Higher Precision: AI models can predict the outcome of genetic interventions with greater accuracy, leading to more reliable and reproducible results in synthetic biology experiments.
- Scalability: AI facilitates the scaling of synthetic biology solutions from lab-scale experiments to large-scale industrial applications, such as the production of bio-based chemicals or sustainable energy sources.
"AI does not only enhance the speed of discovery in synthetic biology, but it also transforms the scope of what is possible–allowing for the creation of highly specialized biological systems that can address global challenges."
Key Challenges and Future Outlook
Despite its immense potential, the integration of AI in synthetic biology still faces some challenges, such as data quality, the complexity of biological systems, and ethical considerations. However, with advancements in AI algorithms and an increasing amount of biological data, the future of AI-driven synthetic biology looks promising, offering solutions for sustainable development, healthcare, and environmental preservation.
Application | AI Benefit |
---|---|
Genetic Engineering | Improved accuracy in designing genes and synthetic pathways |
Metabolic Optimization | More efficient production of biofuels and pharmaceuticals |
Laboratory Automation | Reduction of human error and faster experiment throughput |
Streamlining Genetic Engineering: AI's Role in Accelerating Design
Genetic engineering has evolved significantly with advancements in artificial intelligence (AI), providing researchers with powerful tools to design and optimize genetic sequences faster and more accurately. Traditional methods of gene synthesis and editing are time-consuming, often involving trial and error approaches. However, AI has introduced a new paradigm by automating and enhancing various stages of genetic design, from sequence prediction to gene optimization, thus speeding up the entire process.
AI models, particularly those based on deep learning algorithms, are proving to be crucial in predicting the effects of genetic alterations on biological systems. By simulating genetic changes in silico, AI can identify the most promising genetic constructs before they are tested in the lab, reducing both time and cost. This capability has wide applications, from synthetic biology to drug development and environmental engineering.
Key Benefits of AI in Genetic Engineering
- Speeding up the design cycle: AI can process vast amounts of genetic data and generate optimized sequences in a fraction of the time it would take through conventional methods.
- Improved accuracy: By analyzing patterns in biological data, AI reduces the risk of errors in genetic sequence design.
- Cost reduction: Automating sequence optimization and testing virtually eliminates the need for costly and time-consuming trial-and-error experiments.
AI-Driven Tools in Genetic Engineering
- Sequence Design Algorithms: AI models that predict the best genetic sequences based on specific functional outcomes.
- Gene Editing Optimization: Machine learning algorithms that guide CRISPR and other gene-editing tools to increase precision and minimize off-target effects.
- Biological Pathway Modeling: AI systems that simulate and predict how changes in the genome will affect biological networks.
"AI-powered tools have the potential to revolutionize the field of synthetic biology by accelerating the design and implementation of genetic constructs, making it easier to engineer organisms for a wide range of applications."
Case Study: AI in Yeast Engineering
In a recent study, AI-driven algorithms were used to redesign the genetic makeup of yeast to optimize ethanol production. The AI model not only accelerated the design process but also identified genetic modifications that had previously gone unnoticed. The result was a more efficient production system, with reduced by-products and improved yield.
Modification | Outcome |
---|---|
Gene Knockout | Increased ethanol yield by 15% |
Pathway Optimization | Reduced by-products by 20% |
Promoter Enhancement | Improved metabolic efficiency |
AI-Driven Biosynthesis: Advancing the Production of Bio-Based Materials
Artificial intelligence is revolutionizing the biosynthesis process, enabling the efficient creation of bio-based materials that are integral to various industries. By leveraging AI algorithms, researchers can now predict and optimize metabolic pathways, enhancing the production of valuable compounds such as biofuels, pharmaceuticals, and polymers. AI tools are essential for the design of synthetic biology systems, guiding the engineering of microorganisms for the sustainable production of complex molecules. This leads to higher yields, reduced production costs, and minimized environmental impact.
The integration of machine learning and AI into biosynthesis has made it possible to simulate and analyze biological processes at a scale and speed that was previously unattainable. With AI, scientists can model entire metabolic networks, quickly identify bottlenecks, and suggest modifications that improve efficiency. This not only accelerates the development of bio-based products but also opens up new avenues for innovation in green chemistry and sustainable manufacturing.
Key Benefits of AI in Biosynthesis
- Optimization of Metabolic Pathways: AI-driven models help identify the most efficient routes for synthesizing compounds, reducing waste and increasing productivity.
- Cost Efficiency: AI algorithms lower the cost of research and production by automating processes and reducing the need for costly trial-and-error experimentation.
- Sustainability: AI enhances the ability to design biosynthetic routes that minimize resource consumption and environmental impact.
Applications of AI in Biosynthesis
- Biofuel Production: AI helps optimize microbial strains for efficient conversion of biomass into biofuels.
- Pharmaceuticals: AI aids in the creation of synthetic biological systems that produce complex therapeutic compounds.
- Polymers and Bioplastics: AI is used to engineer microorganisms capable of producing biodegradable plastics and other biopolymers.
AI-Assisted Design of Synthetic Biological Systems
By using AI to simulate and predict biosynthetic pathways, scientists can design more efficient and targeted genetic modifications in microorganisms, making the biosynthesis of high-value compounds both feasible and scalable.
Process | AI Contribution | Impact |
---|---|---|
Pathway Optimization | Machine learning models predict the most efficient metabolic routes | Increased yields and reduced byproduct formation |
Gene Editing | AI tools identify optimal genetic modifications | Faster, more precise genetic engineering |
Production Scaling | AI analyzes large datasets to optimize production conditions | Cost-effective, scalable manufacturing processes |
Automating Laboratory Workflows: The Impact of AI in Bio-Research
The integration of artificial intelligence into biological research has drastically transformed laboratory operations. Automation, powered by AI, streamlines complex workflows, reducing human error and enhancing efficiency. Tasks that once took hours or days to complete, such as data analysis and sample processing, are now handled in a fraction of the time. AI-driven systems enable researchers to focus on hypothesis generation and experimental design, while the AI takes care of repetitive and time-consuming tasks.
AI applications in bio-research go beyond simple automation. These systems are capable of performing predictive modeling, real-time monitoring, and decision-making, offering insights that human researchers may overlook. By analyzing vast datasets, AI tools help identify patterns and anomalies that lead to more accurate conclusions and discoveries. This not only accelerates research but also opens up new possibilities in areas like personalized medicine and synthetic biology.
Key Benefits of AI Automation in Bio-Research
- Increased Efficiency: Automates repetitive tasks, allowing researchers to focus on more creative aspects of research.
- Data Processing: AI algorithms can analyze large datasets much faster than traditional methods, ensuring faster results.
- Consistency and Precision: AI reduces human error, providing more reliable and reproducible results.
- Real-time Analysis: AI systems can monitor experiments in real-time, offering instant feedback and improving the speed of decision-making.
AI in Laboratory Automation: A Practical Example
- Sample Preparation: AI can assist in tasks like cell sorting, liquid handling, and sample dilution, automating tedious lab steps.
- Experimentation: Robots powered by AI perform experiments according to pre-set parameters, ensuring consistent conditions throughout.
- Data Analysis: Machine learning models can interpret experimental results, identify correlations, and predict future outcomes with a high degree of accuracy.
- Reporting: AI systems generate detailed reports, summarizing findings and suggesting areas for further research.
"AI-powered automation reduces the manual labor in laboratories, enabling scientists to move from data collection to discovery at an unprecedented rate."
Challenges and Considerations
Despite its potential, the adoption of AI in laboratory workflows presents several challenges. The high initial costs of implementing AI technologies, coupled with the need for specialized knowledge to operate these systems, can be a barrier for smaller research facilities. Additionally, the reliance on AI algorithms raises concerns about the transparency of decision-making processes and the risk of bias in data interpretation.
Challenges | Impact on Research |
---|---|
High Initial Investment | Increased cost for labs, particularly smaller facilities |
Skill Gap | Need for specialized training to operate AI tools effectively |
Bias in AI Models | Potential for skewed data interpretation and compromised results |
AI-Enhanced Predictive Models in Genetic Engineering
The integration of artificial intelligence into synthetic biology has revolutionized the approach to genetic modifications. Predictive models driven by AI are playing a crucial role in understanding and enhancing the precision of genetic alterations. By analyzing vast amounts of genetic data, these models can foresee the outcomes of specific gene edits with higher accuracy than traditional methods, reducing trial-and-error in laboratory settings.
AI-powered tools leverage machine learning algorithms to recognize patterns and predict the behavior of genetic modifications at a molecular level. This allows researchers to make informed decisions, increasing the likelihood of successful modifications while minimizing undesired side effects. These models not only save time but also push the boundaries of what is possible in gene editing and synthetic biology.
Advantages of AI-Based Predictive Models
- Increased Precision: AI models analyze genetic sequences and predict the impact of changes with remarkable accuracy, helping to avoid unwanted mutations.
- Faster Experimentation: By predicting the results of genetic modifications before they are applied, AI reduces the time required for traditional trial-and-error methods.
- Cost Efficiency: Fewer failed experiments translate to lower costs in research and development, making genetic engineering more accessible.
Applications in Genetic Modifications
- Genome Editing: AI assists in designing more effective CRISPR-Cas9 systems by predicting which guide RNA sequences are most likely to lead to successful gene edits.
- Gene Therapy: AI models predict how specific genetic therapies will behave in a patient’s genome, improving treatment plans for genetic disorders.
- Microbial Engineering: In industrial biotechnology, AI helps predict the effects of genetic changes on microorganisms, optimizing production processes for pharmaceuticals, biofuels, and more.
Key Information
AI models are transforming the landscape of genetic engineering by providing insights that were once unattainable through traditional methods. The ability to predict the outcomes of genetic modifications in silico before they are carried out in the lab is a game changer in the field of synthetic biology.
Example of AI in Action: Predicting Gene-Drug Interactions
Gene | Drug | AI Prediction Accuracy |
---|---|---|
BRCA1 | PARP Inhibitor | 95% |
CFTR | Ivacaftor | 90% |
TP53 | Gene Therapy | 88% |
Enhancing Bio-Manufacturing with AI-Driven Optimization of Metabolic Pathways
Advances in artificial intelligence (AI) are revolutionizing bio-manufacturing by significantly improving the efficiency of metabolic pathways. By leveraging AI algorithms, researchers can analyze and predict optimal gene modifications and enzyme functions, which ultimately lead to higher yields in bio-manufactured products. In the context of metabolic engineering, AI can accelerate the identification of key biochemical reactions that need enhancement, optimizing the flow of metabolites in the system.
AI models, particularly machine learning and deep learning, can rapidly process vast datasets of biological and chemical information. These models can help in predicting the outcomes of altering metabolic pathways, thereby reducing the time and cost traditionally required for experimental trial and error. The integration of AI into metabolic engineering processes is becoming essential for industries like pharmaceuticals, biofuels, and specialty chemicals, where maximizing output efficiency is critical.
AI-Driven Strategies for Metabolic Optimization
- Pathway Design: AI tools can simulate complex metabolic networks, optimizing pathways for specific product synthesis.
- Enzyme Engineering: Machine learning models can identify enzyme variants that improve catalytic efficiency, boosting production rates.
- Gene Expression Optimization: AI can fine-tune the expression of genes involved in metabolic pathways, enhancing overall metabolic flow.
One key factor in improving the efficiency of bio-manufacturing is accurately predicting which genes and enzymes need to be targeted for modification. AI-based solutions are able to model these intricate biological systems with precision, suggesting genetic modifications that will yield the best results in terms of output quantity and quality.
Important Insight: AI significantly reduces the time needed to identify optimal genetic modifications, allowing researchers to focus on refining other variables in bio-manufacturing processes.
AI Implementation in Industry
AI's role in bio-manufacturing has been particularly prominent in the production of biofuels, pharmaceuticals, and other chemical products. The following table outlines how AI is applied across different industries:
Industry | AI Application | Impact on Bio-Manufacturing |
---|---|---|
Biofuels | Optimizing microbial fermentation pathways | Increased ethanol yield and reduced production costs |
Pharmaceuticals | Improving the production of high-value compounds | Enhanced yield and reduced time-to-market |
Chemicals | Designing efficient biosynthesis pathways | Higher chemical yields and cost-effectiveness |
Key Takeaway: AI is essential for optimizing production in a variety of industries, offering both economic and environmental benefits by improving yield and reducing waste.