Ai Voice Recognition Bias
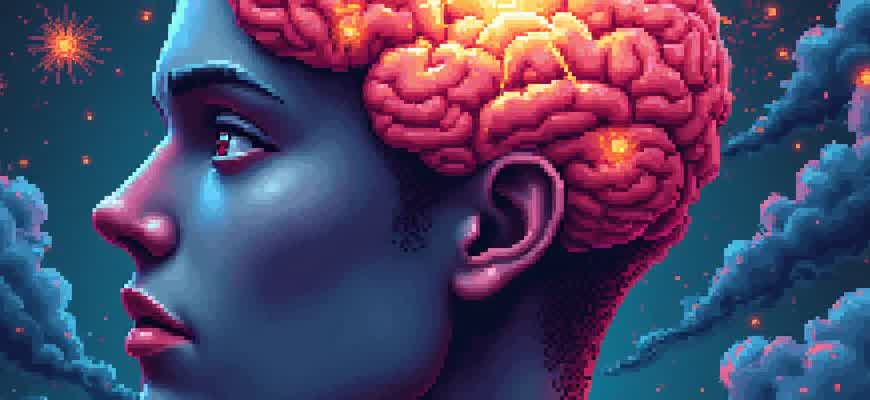
AI voice recognition systems have become integral in various industries, from customer service to virtual assistants. However, these systems are not immune to biases that can affect their accuracy and fairness. One key issue is how voice recognition algorithms may perform unevenly based on factors such as gender, age, accent, and ethnicity.
Factors Contributing to Bias:
- Data Representation: AI models are trained on large datasets, and if these datasets are not diverse, they can reinforce existing biases.
- Algorithmic Design: The underlying structure of AI algorithms may unintentionally prioritize certain linguistic patterns, which could favor specific demographic groups over others.
- Environmental Variables: Background noise, speech clarity, and device quality can also influence the accuracy of voice recognition systems, often disadvantaging certain users.
Consequences of AI Bias in Voice Recognition:
"Bias in AI voice recognition not only undermines the technology's effectiveness but also contributes to inequities in access and opportunities, particularly for marginalized communities."
Group | Common Issue | Impact |
---|---|---|
Women | Underrepresentation in training data | Lower accuracy rates for female voices |
Non-native speakers | Accent recognition problems | Increased chances of misinterpretation |
Minority ethnic groups | Bias towards certain speech patterns | Reduced system reliability and fairness |
AI Voice Recognition Bias: A Detailed Guide
AI voice recognition systems have become integral to many modern technologies, from virtual assistants to security systems. However, these systems are not without their challenges, particularly when it comes to accuracy and fairness. One of the most significant issues is the presence of bias in voice recognition models, which can lead to underperformance for certain groups of people, especially those from diverse linguistic or cultural backgrounds.
The main concern is that voice recognition technologies tend to work less effectively for individuals who do not fit the "average" or most common user profile. This issue can be traced back to biases in the data used to train these systems, as well as the algorithms themselves. When these biases go unaddressed, they can have a real-world impact, such as misinterpretations in voice commands or barriers to access for marginalized communities.
Factors Contributing to Voice Recognition Bias
- Data Imbalance: Most AI voice models are trained on large datasets that may over-represent certain accents, genders, or languages while under-representing others. This can cause the system to perform poorly for those who deviate from the majority.
- Accent and Pronunciation: Many systems struggle with accurately recognizing non-standard accents or pronunciations. These issues can arise from both regional variations and socio-economic factors.
- Language Limitations: Speech recognition tools are often designed for specific languages or dialects, meaning that speakers of minority languages or dialects may face difficulties.
- Gender and Age Disparities: Certain models may show bias towards male voices or voices from younger individuals, as the training data may disproportionately represent these demographics.
Impact of AI Voice Bias on Society
The consequences of bias in voice recognition systems can be far-reaching, impacting both individuals and society as a whole.
- Exclusion of Marginalized Groups: Biases can lead to the exclusion of people who do not conform to the dominant profile, making it harder for them to access certain technologies or services.
- Accuracy Issues in Critical Applications: Voice recognition systems are often used in sensitive areas like healthcare and law enforcement. Inaccurate recognition can lead to serious errors and even safety risks.
- Perpetuation of Stereotypes: When AI systems reinforce biases, they contribute to the perpetuation of harmful stereotypes and further marginalize underrepresented groups.
Solutions and Recommendations
Addressing voice recognition bias requires a multi-faceted approach:
- Inclusive Data Collection: Ensuring that training datasets are diverse and representative of different accents, genders, and languages is crucial in reducing bias.
- Regular Audits and Testing: Conducting frequent audits and testing across different demographic groups helps identify and mitigate bias early in the development process.
- Algorithmic Transparency: Developers should prioritize transparency in their algorithms and make efforts to explain how bias may influence their systems' performance.
Example of Bias in Voice Recognition Systems
Group | Recognition Accuracy |
---|---|
Caucasian Male | 90% |
Black Female | 70% |
Hispanic Elderly | 65% |
"Bias in AI voice recognition systems can be subtle, but its effects are far from trivial. It can lead to misinterpretation of commands, reduce user satisfaction, and even cause unintentional discrimination against specific groups."
Identifying Bias in AI Voice Recognition Systems
AI voice recognition systems have become an integral part of daily life, from virtual assistants to transcription software. However, these technologies often exhibit biases that can impact their accuracy and reliability. Recognizing these biases is crucial to improving fairness and accessibility. Bias in AI systems may arise due to skewed training data, misrepresentation of diverse linguistic features, or algorithmic limitations. Identifying these issues requires a deeper analysis of the systems' performance across different demographic groups and use cases.
Understanding and addressing bias involves examining how voice recognition systems handle various accents, dialects, and speech patterns. Research has shown that these systems often underperform when dealing with non-native speakers, individuals with speech impairments, or people from underrepresented cultural backgrounds. The disparity in recognition accuracy can result in unequal experiences for users, leading to frustration and diminished trust in AI technologies.
Key Factors Contributing to Bias in Voice Recognition
- Training Data Quality: AI systems are trained on datasets that may not fully represent all demographics. For example, if the dataset predominantly features native English speakers from urban areas, it may struggle with rural or non-native accents.
- Speech Variability: Variations in tone, pitch, and speech tempo can affect how well the system recognizes voices. If these features aren't adequately accounted for during training, bias can emerge.
- Algorithm Design: The algorithms powering voice recognition systems may unintentionally favor certain types of speech patterns due to design choices, leading to poorer performance for other patterns.
Steps to Identify Bias in AI Systems
- Test Across Diverse Demographics: Evaluate the performance of the system with users from different age groups, ethnic backgrounds, and speech patterns.
- Monitor Accuracy Variations: Track how the system performs with various accents, dialects, and speech conditions to identify areas of underperformance.
- Conduct User Feedback Surveys: Collect feedback from a wide range of users to assess satisfaction levels and identify common issues related to recognition errors.
Bias in AI voice recognition not only impacts user experience but also raises ethical concerns about fairness and inclusivity in technology. Addressing these biases requires comprehensive testing, more diverse datasets, and transparency in algorithmic decisions.
Examples of Bias in Voice Recognition
Accents/Dialects | Performance Impact |
---|---|
Non-native English accents | Lower recognition accuracy, especially in regional dialects. |
People with speech impairments | Difficulty in accurately interpreting altered speech patterns or vocal nuances. |
Gender differences in pitch | May affect recognition accuracy, with certain systems performing worse with female or higher-pitched voices. |
How Demographic Factors Affect AI Voice Recognition Performance
AI voice recognition systems are increasingly being used in a variety of applications, but their accuracy varies significantly depending on several demographic factors. These factors include age, gender, accent, and ethnicity. The technology has been shown to perform better with certain groups while struggling with others, often leading to disparities in usability and effectiveness. Understanding how these demographics impact the performance of AI systems is essential for improving fairness and inclusivity in voice-based interfaces.
Research has demonstrated that machine learning models for speech recognition are often trained on data that predominantly represents certain accents or speech patterns, which can lead to a lower accuracy for individuals whose voices fall outside the norm of the training data. This bias can affect how well these systems understand and process speech from different groups, highlighting the need for more diverse training datasets to address these gaps.
Key Demographic Influences
- Gender: Voice recognition systems may perform differently based on the gender of the speaker. Historically, systems have been optimized for male voices, leading to higher accuracy rates when recognizing male speech compared to female voices.
- Age: Older individuals often face challenges with AI voice recognition due to changes in speech patterns associated with aging, such as slower speech or more pronounced accents.
- Accent: AI systems may struggle with regional accents or non-native pronunciations. This is particularly true for systems trained primarily on standard accents like American English.
- Ethnicity: Voice recognition technologies may be biased against certain ethnic groups if the training data lacks representation of their speech patterns, leading to lower recognition rates.
Impact of Demographics on AI Accuracy
Demographic Factor | Impact on Recognition Accuracy |
---|---|
Gender | Higher accuracy for male voices due to overrepresentation in training data. |
Age | Lower accuracy for older individuals as speech changes with age. |
Accent | Decreased accuracy for non-standard or regional accents. |
Ethnicity | Bias toward dominant ethnic speech patterns, leading to errors for underrepresented groups. |
"The lack of diverse representation in training data has a direct correlation with the performance gaps observed across different demographic groups."
Understanding the Impact of Accent and Dialect on AI Systems
Voice recognition technologies are becoming an essential part of daily life, from personal assistants to customer service automation. However, these systems often face challenges in accurately processing the diverse range of accents and dialects spoken by individuals around the world. This issue highlights the limitations and biases present in current AI voice recognition systems, which are primarily designed to understand standard accents or specific regional dialects. As a result, users with non-standard accents may experience reduced functionality and accuracy, leading to frustration and inequality in user experience.
AI systems that rely on voice recognition often struggle with understanding regional pronunciations, speech patterns, and colloquial phrases. This is particularly evident in multilingual societies or countries where accents vary widely even within a single language. If these systems are not trained to recognize the nuances of different dialects, they may misinterpret commands, fail to transcribe accurately, or simply not work at all for certain individuals. This inconsistency raises concerns regarding fairness and accessibility, especially for those with less mainstream accents.
Factors Contributing to Voice Recognition Bias
- Lack of Diverse Training Data: Many AI systems are trained on data that predominantly includes mainstream accents, which limits their ability to understand less common speech patterns.
- Accent Stereotyping: Some systems may be overly optimized to recognize specific accents, causing them to overlook others entirely.
- Regional Dialect Variability: Even within the same language, different regions may have vastly different ways of speaking, making it difficult for AI to identify and process all variations accurately.
"The accuracy of voice recognition depends heavily on the quality and diversity of the dataset used to train the AI. If the training set is skewed toward a particular accent or dialect, the system may perform poorly for users with less common speech patterns."
Challenges for Non-Standard Accents
- Pronunciation Variance: Different pronunciations of the same word can confuse voice recognition systems that are not equipped to handle such diversity.
- Phonetic Differences: AI systems may not be able to differentiate between phonetic shifts that occur in various regional accents, leading to errors in speech recognition.
- Cultural Context and Idioms: Certain dialects may include cultural references or idiomatic expressions that voice recognition systems are not trained to understand.
Addressing Bias in AI Voice Recognition
Approach | Impact |
---|---|
Expanding Training Datasets | Incorporating diverse accents and dialects into training data improves recognition accuracy across various speech patterns. |
Localized AI Development | Creating region-specific models ensures that voice recognition systems are better attuned to local speech variations. |
Continuous Feedback and Refinement | Gathering user feedback on recognition errors helps developers refine the system's ability to understand diverse accents over time. |
Data Quality: Key Factor in Reducing Voice Recognition Bias
In the development of voice recognition systems, the quality of data used for training plays a critical role in minimizing bias. High-quality data ensures that the system is capable of accurately recognizing diverse accents, dialects, and speech patterns. When the training dataset is incomplete or unbalanced, the model may struggle to understand voices that differ from those it was primarily exposed to. This can lead to misinterpretations and reinforce existing biases in the technology.
Improving data quality requires careful selection, diverse representation, and constant updates to the training datasets. Moreover, it involves eliminating errors and inconsistencies that may skew the system's performance. It is not enough to just collect more data; it must be representative, balanced, and carefully curated to achieve fairness and accuracy in voice recognition systems.
Key Elements of High-Quality Data
- Diversity of Speakers: Ensuring that data includes a broad spectrum of voices from various demographics such as gender, age, ethnicity, and geographic location.
- Accurate Transcriptions: Data must include precise transcriptions to avoid errors in speech-to-text conversion and subsequent bias in model predictions.
- Contextual Variability: Including different environments and situations in which speech occurs, such as noisy backgrounds or varying emotional tones, helps the system handle real-world scenarios.
Approaches to Enhancing Data Quality
- Expanding Data Sources: Gathering data from underrepresented groups and regions to ensure the system is not biased toward any specific group.
- Regular Data Audits: Continuously evaluating and cleaning datasets to remove errors or outdated examples that may reduce the model's accuracy over time.
- Incorporating User Feedback: Using real-world interactions to refine and improve the system, helping to identify areas where the model may underperform or misinterpret speech.
By improving the data quality, voice recognition systems can become more inclusive, accurate, and reliable across diverse user populations.
Impact of Data Quality on Bias Reduction
Factor | Impact on Bias |
---|---|
Diversity in Training Data | Helps reduce bias by ensuring the system learns from a wide range of voices and accents. |
Accurate Transcriptions | Minimizes errors that can introduce bias into speech recognition results. |
Contextual Variation | Improves model adaptability to real-world conditions, reducing the risk of biased performance in specific environments. |
AI Voice Recognition in Diverse Languages: Overcoming Challenges
Voice recognition systems have become integral in everyday life, from virtual assistants to customer service automation. However, one significant issue that arises is their performance across various languages, especially when it comes to underrepresented or diverse linguistic groups. Many current AI systems are trained primarily on data from dominant languages, leading to lower accuracy and efficiency when processing speech from speakers of minority or regional languages.
The main challenge is the data imbalance in training sets, which often lack diversity in accents, dialects, and speech patterns. As a result, speech recognition systems can show biases, such as misinterpreting non-native accents or failing to recognize specific linguistic structures, making it difficult for people from diverse backgrounds to fully benefit from AI-driven voice technology.
Key Challenges in Multi-Language AI Voice Recognition
- Data Availability: Many languages, especially minority ones, lack sufficient speech data to train AI models effectively.
- Accent and Dialect Variation: Accents and regional dialects complicate recognition, leading to misinterpretations of speech.
- Cultural Context: AI may fail to understand cultural nuances embedded in language use, affecting both accuracy and user satisfaction.
Strategies for Overcoming These Challenges
- Expanding Data Sets: Increasing the diversity of speech data by including more voices from different regions, accents, and dialects can enhance accuracy.
- Localized Training: Tailoring AI models to specific linguistic groups through localized datasets helps recognize regional speech patterns more effectively.
- Incorporating Contextual Understanding: AI systems can be enhanced to understand context and cultural subtleties, improving overall performance in diverse linguistic settings.
"Improving voice recognition technology for underrepresented languages is crucial for ensuring equitable access to AI-driven services."
Performance Comparison: Languages with High vs Low Representation in AI Models
Language | Representation in Training Data | Recognition Accuracy |
---|---|---|
English | High | 90% |
Mandarin | High | 85% |
Swahili | Low | 65% |
Quechua | Very Low | 50% |
Ethical Concerns: Addressing Discrimination in AI Voice Technology
AI voice recognition systems are becoming integral to many aspects of daily life, from virtual assistants to customer service. However, concerns have emerged regarding the potential for bias in these technologies, which can lead to unequal treatment based on factors such as accent, gender, or ethnicity. Discriminatory outcomes are especially prevalent when these systems are trained on datasets that lack diversity, resulting in reduced accuracy for certain demographic groups.
As AI systems rely heavily on voice data to make decisions, they can inadvertently reinforce stereotypes or marginalize certain groups. This issue raises important ethical questions about fairness, accountability, and transparency in AI development. The challenge lies in creating technology that is both inclusive and unbiased, ensuring equal treatment for all users regardless of their background.
Key Ethical Issues in AI Voice Technology
- Bias in Speech Recognition: Systems often struggle to accurately interpret voices with non-standard accents, leading to errors and frustration for users who do not fit the "norm" represented in training datasets.
- Exclusion of Marginalized Groups: Certain accents, dialects, or speech patterns may be underrepresented in datasets, leading to the marginalization of specific communities.
- Discriminatory Outcomes: Inaccurate voice recognition can result in poor user experiences or even reinforce negative stereotypes, especially in applications related to security and authentication.
Approaches to Mitigating Bias
- Inclusive Datasets: Ensuring that AI models are trained on diverse datasets that represent a wide range of accents, languages, and dialects is crucial to mitigating bias.
- Transparent Development Processes: Developers should make their processes open and accountable to external scrutiny, allowing third-party reviews of models for fairness.
- Continuous Testing and Feedback: Regular testing of voice recognition systems with real-world users, followed by incorporating feedback, can help identify and rectify bias.
Impact of Discrimination in AI Voice Technology
Issue | Potential Consequences |
---|---|
Inaccurate Recognition of Non-Standard Accents | Decreased user satisfaction, increased frustration, and potential exclusion from technology access. |
Reinforcement of Negative Stereotypes | Perpetuation of harmful societal biases, affecting the way certain groups are perceived and treated. |
Unfair Authentication Systems | Denial of access to services, such as banking or security systems, based on voice misinterpretation. |
Quote: "AI technologies must be developed and deployed in a way that ensures equity for all users. Bias in voice recognition not only impacts individual users but can also contribute to broader social inequalities."
Practical Steps for Businesses to Minimize Bias in AI Systems
Bias in AI systems, especially in voice recognition technologies, can lead to significant challenges in customer interactions, inclusivity, and brand reputation. As businesses increasingly integrate AI for various functions, from customer service to product development, it's crucial to implement steps that ensure fairness and reduce biases embedded in these systems. Addressing bias is not just a technical issue but also a responsibility to provide equal and inclusive experiences for all users.
By proactively tackling bias, companies can improve both the accuracy and ethical standards of their AI systems. Below are practical measures businesses can take to reduce bias in AI-driven voice recognition technologies.
Key Actions to Reduce Bias in AI Systems
- Improve Training Data Diversity: Ensure that voice recognition models are trained on diverse datasets, including various accents, dialects, and speech patterns. This helps prevent the AI from being overly optimized for a particular group.
- Regular Model Audits: Continuously assess AI systems for biased outcomes. This includes testing performance across different demographic groups to identify and correct discrepancies.
- Inclusive Design Practices: Involve diverse teams in the development process to reduce unconscious bias and ensure that different perspectives are considered throughout the design stages.
- Transparency and Accountability: Make the decision-making processes of AI systems more transparent, allowing for external scrutiny and validation. This can help identify any underlying bias in algorithmic outcomes.
Steps for Continuous Improvement
- Establish Bias Detection Tools: Use specialized software to monitor AI performance and flag potential biases during operation.
- Collaborate with Diverse Experts: Work with linguists, sociologists, and other experts to evaluate the inclusivity of your AI systems, ensuring cultural and regional differences are well-represented.
- Empower End-Users: Enable users to provide feedback on AI interactions. This can help businesses understand potential biases and address them quickly.
“By taking proactive steps to ensure fairness and reduce bias in AI, businesses can build trust with their customers, improve system accuracy, and foster an inclusive digital environment.”
Summary of Action Plan
Action | Benefit |
---|---|
Improving Data Diversity | Ensures better accuracy across various demographic groups. |
Regular Audits | Helps identify and rectify biases in AI models. |
Inclusive Design Practices | Reduces the risk of systemic bias in voice recognition systems. |
Transparency | Builds trust and accountability among users. |